Episode 4: Have Classification Algorithms Gone Too Far? Exploring Gender in AI
Featuring Morgan Klaus Scheuerman
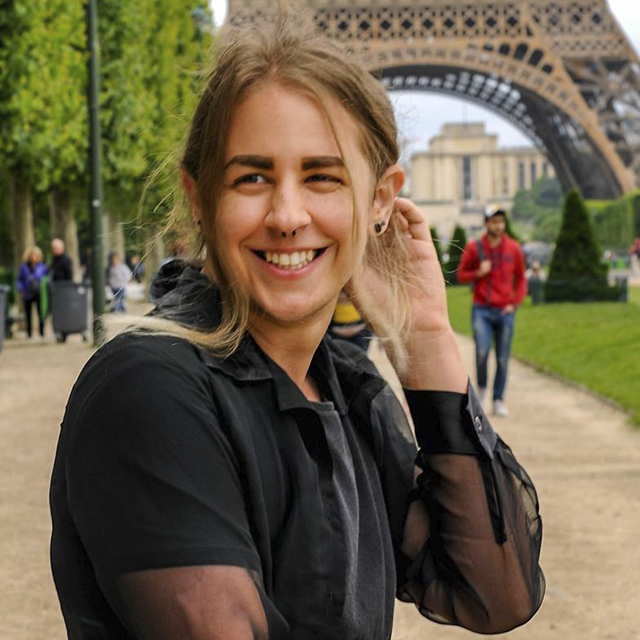
In this episode of the Radical AI podcast hosts Dylan and Jess interview Morgan Klaus Scheuerman.
Morgan is an Information Science PhD student at the University of Colorado interested in exploring the ways individuals with diverse gender identities interact with technology. He grew up in Maryland, where he earned a Bachelor of Art in Communication & Media Studies with a minor in Gender Studies at Goucher College and a Master of Science in Human-Centered Computing at University of Maryland, Baltimore County. His master thesis work focused on the way transgender individuals' experience safety and bias when interacting with digital technologies. At CU, he works with Jed Brubaker in the Identity Lab. In his spare time, Morgan enjoys travel, hiking, photography and consuming snobby hipster coffee beverages in mid-century modern cafes.
You can follow Morgan on Twitter at: @morganklauss
4. Morgan Klaus Scheuerman transcript powered by Sonix—easily convert your audio to text with Sonix.
4. Morgan Klaus Scheuerman was automatically transcribed by Sonix with the latest audio-to-text algorithms. This transcript may contain errors. Sonix is the best audio automated transcription service in 2020. Our automated transcription algorithms works with many of the popular audio file formats.
Welcome to Radical a-I, a podcast about radical ideas, radical people and radical stories at the intersection of ethics and artificial intelligence. We are your co-hosts, Dylan and Jess. Just as a reminder for all of our episodes, while we love interviewing people who fall far from the norm and interrogating radical ideas, we do not necessarily endorse the views of our guests on this show.
We encourage you to engage with the topics introduced in these conversations and to take some time to connect with where you stand with these radical ideas. We also encourage you to share your thoughts online on Twitter at Radical. A I pod.
In this episode we interview Morgan Clough's Schwieterman. Morgan is an information science p_h_d_ student interested in exploring the ways individuals with diverse gender identities interact with technology, with a focus on the way that transgender individuals experience safety and bias when interacting with digital technologies.
As always, as a sneak peek of the interview that will follow, we will begin this episode with a segment that we like to call Loved, Learned or Leave, where we discuss some of the major topics brought up by our guest that we loved, that we learned and any topics that we might want to leave behind. By that we mean topics that may have challenged us during the interview. For me, one of the things that I loved about this interview with Morgan was his interdisciplinary lens. Morgan really brings together art, communications, computer science and so much more into his understanding of what's important and vital in the conversation of artificial intelligence ethics. Morgan also straddles this space between the academy and the industry, which right now is so important for all of us to do as we discern what artificial intelligence ethics really looks like out in the fields. One of the things that I learned from Morgan is more about how classification systems work, which is something that I really have very little experience in. And I also learned a bit about the disconnect between the companies that are creating these classification systems and the consumers that are then using those systems. Which brings me to the thing that I would leave behind, which in my notes from the interview was I want to leave behind capitalism, which is its kind of broad. But what I mean by that is I think that we need to interrogate some of these systems that are creating a disconnect between the industry and between the consumers. Part of that has to do with representation and part of that has to do with how we build our systems. Part of that has to do with data.
But I think there's a bigger critique here that Morgan talks about a little bit, maybe not as explicitly as I'm talking about right now. When I just mentioned capitalism in bold font, but I think there's something there that really challenges me of how do we work within this capitalist capitalistic system while still creating artificial intelligence ethics across the board. Yeah, let's just leave behind capitalism. Let's do it. Who needs it? That's what I'm saying.
So something that I really loved about this podcast was actually the same thing that Dylan loved about this podcast. And that's how inter-disciplinary Morgan's work as. He does a really great job of bringing in his background in women and gender studies into this notion of algorithmic identity. And one of the ways that he did this that was really great for me to see that I haven't really thought about before is in this identity space, especially in this gender space. When we talk to people who come from a computer science background like myself, we tend to think of gender as something that's intuitive. And a lot of people tend to think of gender as intuitively male or female. This binary idea sort of like a computer would. And then you talk to somebody like Morgan who comes from this woman and gender studies background and he thinks of gender as this squishy area and so do a lot of people in that field. And that's not something I've really thought of all that much myself. So I think that just sort of leads to this important notion that we really need to have diverse minds and diverse backgrounds in these spaces when we're designing these algorithms. And speaking of identity in this squishy space, something that I learned that Morgan mentions at a certain point in the episode is what he believes identity to really mean. And he talks about the idea of. Entity as being something that is self-proclaimed.
That is something that you create and define for yourself and something that a computer would do or something. Shoot a little. OK. Mm hmm. I know. I know. That's OK. Yeah. I'll just start with the learned again.
And something that I learned was this notion of identity versus identification. And Morgan does a really good job of highlighting this and how he believes identity is defined. So he thinks of identity as something that is self-proclaimed. So you define your own identity. But when it comes to a computer, especially in these classification softwares and algorithms, that is an identification of a person. So a computer can not proclaim someone's identity, but they can identify someone. That was really interesting for me. I've never really thought about that before and something that I would leave behind. So something that challenged me. This is actually something that I entirely agree with. But it's challenged me a bit in the past. It's this idea that there is no way to unbiased a system entirely because there will always be bias in algorithms, just as there will always be bias in their designers because we're human. This is something that has been particularly challenging for me to come to terms with in my own research, because a lot of my research is basically how do we take the bias out of algorithms. And so coming to terms with the fact that that isn't going to be completely possible, is it a little bit disheartening sometimes. But I think it's also realistic and it's important to stay pragmatic about these ideas because we can't actually solve societal problems through algorithms and through technology, at least in all of the situations that I've encountered so far. If anyone finds a situation where we can solve a societal problem through an algorithm, please let me know because I'd love to do some research on it. But I think that this is just something that is really challenging for not only me, but for a lot of people in academia and in industry. The designers of these algorithms and the people who are influenced by these algorithms to here is that there will always be bias, there will always be unfairness in these algorithms. So how do we find the way to mitigate this bias and this unfairness as best as we can?
Well, just as you know, bias and fairness are themes at the heart of this show. But what would be really unfair is us withholding this interview with Morgan any longer. We are so excited to share this interview with you all.
Well, Morgan, it's great to have you on the show. Thanks for coming and joining us. We would love to just get started by getting to know you and who you are a little bit better. So if you'd like to maybe just give us a little bit of your backstory where you've come from and what has brought you to where you are today.
Yeah, thanks for having me on the show. It's great to be here. So I am currently a PFC student in Information Science at University of Colorado, Boulder.
And there I study kind of broadly how identity is represented in algorithmic infrastructures. Primarily I've been looking at computer vision specifically, so things like facial analysis and facial recognition and how both gender and race are represented in those infrastructures. Prior to starting my p._h._d. I did a masters in human centered computing that was really more focused on the design side of this space. This kind of broad human computer interaction space. And there actually got started in research on kind of accessibility, especially for people who are blind or visually impaired and how they interact with technology and how technology can provide them kind of information about the world that might otherwise be inaccessible. And so I was doing a project with some p_h_d_ students there who whose primary research was in this area. And so we were kind of exploring how people who are blind navigate interpersonal interactions with people, particularly. Instances where they might not feel as safe just either walking around or in like a city. And so we were exploring how computer vision could offer kind of safety information for people who are blind to make determinations about other people. And so this actually is what kind of sparked my interest in how computers classify people visually. And so that's when I decided, I guess a lot of things made me decide to do a p._h._d. But when I decided to do a PDG, I knew this was the area that I wanted to research.
Very cool. So was this like in terms of like technology, ethics and an identity? Were these always things that you were interested in, like growing up or is this like a pretty new thing that you drove into?
Yeah. You know, I don't think this is actually something I thought that much about growing up. I mean. I I guess as maybe a millennial, I grew up not entirely with technology, but once I hit the middle school high school years, I I used computers, at least desktop computers regularly. But I never really thought about how they were designed, particularly in terms of like who is designing them for what they were designing them and for who they were designing them for. Right. So actually, I think I never really start to think that critically about this until. I guess probably until I went to do my bachelors, which was in communication, which wasn't a major, it wasn't human computer interaction. But there was some focus on telecommunication technologies. And so I really got my first look into what human computer action was from that. And so actually I read Danah Boyd and one of my classes, and I think that was my first like. Introduction to this idea that, oh, humans are designing things and how they design things impacts the way they are used. And so and also I did a minor in women gender sexuality studies, and and we didn't again, we didn't really talk about technology in those spaces either. I don't I still don't know how often people focus on technology. That's not traditional media in those kinds of spaces. But it also kind of opened my eyes to, you know, histories of inequality or not having access to the same things depending on your identity. And so I think a synthesis of those kind of to. Learning area for me when I was in college kind of led me towards this route. But even then, I don't think it was until my masters where I really started thinking about like researching that.
Yeah.
I'm wondering for folks who are maybe new to the high ethics landscape, who don't know a lot about it, but what the big issues of identity are or the big issues of fairness are out in the world. If you could kind of just give a brief primer to some of the things that you're looking at specifically and maybe talking a little bit about like what's at stake in some of these questions.
Yeah. So I guess fairness is becoming more of a research area. And I recently I mean, I'm sure there have always been people who have been thinking about this. I mean, there's there are pieces from Helen Nissenbaum from like the 90s, early 2000s thinking about bias in machines. But as an area of focus and like a major research area, I think fairness is relatively new. And it's also relatively nebulous. What that means, because it means a lot of things for a lot of different people. So for some people, fairness is just kind of like equal opportunity or equal output for the four different types of people and for others. Fairness is maybe more of this like social sciencey type of how are we, how we embedding the reality of, you know, differential. Access into these systems, so maybe thinking less about metrics and more about the experiences people have historically had off either off line or not with technology. And again, in technology. And so that's kind of maybe for me more where I'm thinking about fairness is kind of like what are the social systems our technologies operate in? And when I'm thinking about identity, which is as equally a nebulous term, I'm actually thinking a lot about this tension between kind of, you know, when you're identifying yourself to me, you have this idea of who you are, right? Some kind of self held subjective notion of who you are. But I have a different notion. Right. Because I have a different perspective. And so I am thinking of identity as like where someone self held internal identity meets a computer's like. Determination based on what it's taught. So more of an identification of someone. And so those are kind of the lines I've drawn for identity because they're you know, the further I get into this, the more I realize that there are like dozens and dozens of theories of what identity even is. I'm not sure that helped.
Definitely. And speaking of identity and computers identifying there is it seems like the term that's usually used for this process is classification. And I know that a lot of your research has to do with A.I. classification. So maybe you could speak a little bit to what that even is like, how it works and how that influences your work in this realm of fairness and equity.
Yeah. So classification, I'd say, in terms of computer vision usually means the computer is kind of supplied a set of labels that it can choose from when it's classifying something about a person. And based on basically the history of data, it's been given to train and. It will make a decision. It can't make new classifications that can only put people into those. Right. And so. I guess I've been thinking a lot about what the limitations of the classifications are often because. The people who are designing the systems, whether they be companies or machine learning researchers who are also, you know, p_h_d_ students or faculty like myself. I think the the way that they've been trained is much different than the way that I've been trained. And so I'm trying to kind of synthesize like, oh, they're they're sort of trained to see the world of classification as kind of this obvious thing. Like it's just.
Very intuitive.
So, like, I guess I I'm trying to build a system I know I wanted to classify gender when I think about that intuitively. It's male and female, right. Because that's how, you know, I've been taught to view things maybe in computer science. I've never really taken a class that's dealt with gender as a theory or like a weird, squishy area, whereas I'm coming from a background that's kind of talked a lot about these weird, squishy areas. And so I'm kind of using my background as a way to.
View a totally different epistemology, I guess.
I don't remember 100 percent what your question was or if I answered the question.
No, I absolutely I think that. So I resonate a lot with with what you just said, because I'm coming from a sociology background and a religious studies background and I have some technical background, but but not so much.
And it's interesting to me coming into a field where there seems to be so much of a need for certainty and like categorizing things as in buckets. Right. So even if we moved the buckets around or make them bigger buckets, there's still this need for data sets to fall into certain buckets.
And I have noticed that my expertise and say moral philosophy can ask like simple questions that alter how we might view those classifications. And I'm wondering for you how you navigate that interdisciplinary world that you're straddling.
Yeah. I mean, I think it's difficult because I think there are many ways to approach it. If you're an interdisciplinary researcher and the way that I want to approach it is both from this pragmatic perspective that really matters to the people who are building systems and also from this kind of critical theory lens that questions those systems in the first place. But at the same time, I want to meet in the middle because I want I know that these systems will exist regardless of what some critical theorists will say about them. And so I want to kind of push the field a little bit in maybe what maybe what you'd call a more radical direction. Right. And so I try to. I mean, it's easy to go down this rabbit hole of like, well, maybe the classification system shouldn't exist, but that's also not realistic in a in any technical or feasible way. So instead of arguing that the tech classification system shouldn't exist, we should maybe think more about the social reality that classification system is enforcing. What use cases are specific domains? Is it going to kind of exert its classification in and how can we rethink classifications in kind of a contextual way? So maybe in advertising it should be different than insecurity. So I'm trying to think of basically use it using that that critical theory kind of weirdness background as a lens more than the final argument or the final output of my research.
And when you say radical in terms of pushing it in a radical direction, can you impact that a little bit about like what you mean by that? And in what way is that your researcher or maybe even your identity in this field is radical in that way?
Yeah. So I've actually been thinking a little more about what radical means since basically since you all asked me to write it. What radical is in your questions? So I think radical is one of those double edge like a double edged sword type of term. So on one hand, it can imply to people like kind of this unrealistic or extremist view, which I don't I personally don't agree with that side of it. But if you're coming from like a totally different background and somebody is proposing an idea that seems really radical or really extreme, maybe it does come across that way. So but what I'm thinking about, maybe radical, I'm just thinking about changing the status quo of a field or at least maybe insidiously. Putting certain ideas in the minds of people who might not otherwise be exposed to it. So.
I guess.
An example of kind of a radical idea for me would be like, well, this field has I mean, it's been around since like really the 40s. I think the 60s is when image recognition became more of a thing, but it's been around for a while. But it's also relatively young, right. But it has its established kind of view of the world. That says, like, you know, we have X number of races that we can classify, we have X numbers of genders that we can classify. And in my work recently, I've noticed that there's not a lot of questioning of that. And so I think to me, a radical idea is even just pushing a little bit in the direction of like, well, can we question what race means in the context of our our technological system just because it doesn't seem radical, maybe from the perspective of like philosophy. But it is radical in that this field isn't doing that very much. So just kind of changing the status quo a little bit would be radical from that perspective.
Yeah, and going along with that same idea. What do you think about you and who you are as a researcher and where you come from makes you possibly radical in this space?
And what helps you do the work that you do? Yeah. That's a good question.
That's maybe one of the harder questions for me. I mean, I guess I feel personally, I feel like any person, whether they're a researcher or a designer or a an engineer, brings some aspect of who they are to the table when they're doing whatever work they're doing. So I believe in this concept of like kind of you have a standpoint. So like feminist theory has this concept of standpoint theory where your background is influencing everything you do. So I really think there's that my background in I don't know, I have I have a very like weird windy background where I was initially in the arts and then I moved into communication and I decided I don't know why I decided to take gender studies, but I did. And so I took that. And that really opened my eyes to even how like science broadly, like medical science, like hard science has been heavily influenced by, you know, race and gender and and both of those things in an interconnected way. And so I think. That has really been the reason that I've really focused like why I feel like. Questions that I ask seem really obvious to me. And then they aren't obvious to other people, which it feels really weird because it feels like, oh, I'm asking this very obvious question, like why has no one asked why male and female are the categories used in gender classification in this field? Which seems like an obvious question, but there has been no work actually examining that. So there had been no research that had empirically examined like. How those classifications are actually impacting different people. And so I think just being able to ask this very simple question helps. Me kind of carve a path that hadn't been examined before in this field.
Why is it so important for especially, I'm thinking, companies who are designing these algorithms? Why is it so important for them to ask some of those questions about classifiers like again, like what's what's really at stake there? Downstream.
Yeah, I mean, I think there are multiple answers for multiple stakeholders, right? I think the most obvious answer that people seem to care about in terms of like we're building a system we wanted to play it for as many people as possible is. PR, which is like not the most exciting or socially like.
I don't know. It's not it's not the most exciting or lake. Sorry, I'm searching for a word.
So it's not the most exciting or like socially aware answer, but a lot of companies have. Faced some backlash for not thinking about these things, particularly, I think, in terms of race. You know, there are a lot of stories out there about like. Mis classifications of race being really offensive or mis classifications of cultural norms like what a wedding looks like in a different country being really offensive because a company's classifier got it wrong. And that. That kind of bad PR just doesn't look good for the company, they're afraid of losing money. And so that's one kind of motivator for them. And another motivator is obviously just like if they have a market that they want to reach and it's worthwhile, can they reach that market? So. I would say on the other side, there's kind of the social responsibility and the and the perspective of citizens. So like people are concerned about classification system as being flawed or biased or just not recognizing them because they might have to interact with it, they they might also not even be aware they're interacting with it, which makes it kind of this weird surveillance thing that. It could be even more concerning. So, I mean, I did a research study where I interviewed transgender and non-binary people about their perspectives on gender classification and how generally it is a binary classification system. And most of them were concerned as citizens, right. So they didn't want to interact with such a system and they were concerned about how certain parties might use it. So particularly governments and governments. And that's, you know, linked to social histories of being discriminated against.
Yeah. Well, you've mentioned before to us that in your research you have worked not necessarily with the government, but with some of these big corporations and big tech companies in industry. And because they are the ones who are coming out with these different facial classification softwares and using them on the public, whether or not the public is aware of this.
You've kind of opened up a little bit of a Pandora's box in some ways to for some of these industry people to kind of see the effects of what they're doing. So I'm curious what your experience has been working with industry and having this dialogue between your research and the output of what they're creating, what that's been like for you, if there's been any pushback or if it's been smooth sailing? What what does that feel like?
Yeah. So I guess in this context, I won't talk specifically about any certain companies, but I think I've gotten a little bit of both. I mean, not necessarily pushback or any kind of argumentative stance as much so much as like we're going to defend our product. So I've definitely had that. And then on the other hand, I've also had a company that reached out to basically a team and a company not like the whole company obviously reached out to work on improving this for their system. So I would say that, you know, it really depends on like what a team is working on, who's reaching out to. Right. So the person here reached out to kind of defend the product was a PR person, the person who reached out to work on their product as an engineer. So I definitely think that at least even when they're pushing back, there's kind of this nice opportunity to make them. Oh, wait. Like somebody is looking at this. We maybe haven't thought about it or maybe we have them. Maybe we should reassess it. So there is at least this little like wedge that you can, you know, push on and make them rethink maybe some of their systems. And then there's always this potential opportunity that someone on a team will see you as a potential expert or potential benefit to that team. So you can actually, like tangibly improve how they're doing things, which is really cool.
I have a question about bias and fairness and classification and all of that, which is kind of the. What do we do? Question, which is that like so there's kind of two two modes of thought about bias that that I've experience, one being that we need to continue. If we keep working, if we keep making our technology better and our classification systems better and everything better with more technology, then we will be able to solve bias and prejudice in technology, including in facial recognition technology.
So that's school.
And then school, too, is basically saying we're always gonna have some level of bias because the classifications that we're using are inherently flawed and have bias embedded within them. In both of these, especially in the second one, it seems like we kind of just do the best we can with what we have. And it's always going to be this, you know, chasing the carrot, as it were. But like what? What do we do? And do you have a vision for what classifiers can look like or should look like, say, in like 20 years if there is like an ideal classifier?
Yeah. So I will say, like, first off, I disagree with school one. I actually really do not believe there's any way to un bias a system entirely because. Well, first of all, bias isn't necessarily a bad term, right. Like it could be that it's trying to think of an example where it's not a bad term, but like maybe there are some systems that you want to prioritize, like at risk groups. And that's a bias, but it's a good bias. And because the systems are reflective of their human graders, there will always be bias, which sounds kind of hopeless. But I think it's a reality that really needs to be acknowledged because there is no to me no utopian end of bias. We as humans would no longer be bias then. In terms of. An ideal classifier, I guess I've never really thought of it because I think, again, all classifiers are going to be limited in some way. And also the more that I've delved into like, well, what do we actually do if this system is flawed in terms of like recognizing gender, right. Do we just add another gender? Well, unfortunately, that technically doesn't really work because the reason that they're flawed in terms of gender is that the very like notion in the very argument from maybe a feminist or trends theory perspective is that you can't tell gender from looking at someone.
Right. And so just adding a third option isn't going to make that classifier work any better. It will probably actually make it work much worse for like the binary CIS gender men and women who interact with it, because then there's this kind of middle ground where it's like, well, it could look like anything. Right. And it also is a little wonky in terms of race for that reason. But it's easier for people to bucket their training data into race and just be like, well, this person is this race and this person is that race. And if they look like that, that's what they're gonna look like. But again, if you have a race classifier, you're always going to leave out some race or you're always going to forest someone's maybe mixed race status into a bucket of only one of those races or just this like other bucket race. And so I don't actually see any. Future for like a perfect classification system. But I think classification systems can be useful for certain use cases. So say you want to like measure. Like the bias of, like, I don't know, animated female characters in children's TV, so maybe you want to be able to at least recognize like someone, some character looks feminine. And so you can classify that and maybe see like they have X percent of main character roles or X percent of scream time.
And then I think actually the best way to use a classifier is to also use it in tandem with like human decision making. So how can you then cross-reference like this list of classifications in children's TV shows with. You know, scrips or what you actually know about the character or what has been confirmed about the character. Because I can see there might be some outliers in that. Right. So like. An outlier that I always go to when I use this example is in she RA. They now have this like non-binary character. And so your classification might get that wrong. But you can actually cross-reference that. It's a little more labor. It's not as automated it. But I think if you have if you're using classification for these kind of micro problems to solve little tasks that are actually really useful for us as people to understand a larger issue, then I think it's appropriate. I think for me what I really want to see more of in how we're using these is an acknowledgement of the limitations and an acknowledgement of like the researchers perspective or the engineer's perspective, like where are they coming from when they're making this system? What decisions and tradeoffs are they making in terms of like what we're going to classify? And sadly, that is not present. And that is part of the issue.
Yeah, I think that's something that we see a lot today when it comes to these issues of like ethical problems with different A.I. systems is we need to have some sort of acknowledgement to the general public, especially to people who aren't really aware of how these systems work, like we need to let them know and build awareness that there are these limitations so that people understand what's happening and they don't just place too much trust in these machines and they don't just assume that it's always acting in the most ethical way. So I'm curious, like with that idea in mind and with your experience in the past, like working with people in industry, but then also having your your work be grounded in academia. What do you think is the right path or maybe what do you think could be a good path in terms of responsibility and like building this awareness going forward? Who do you think is responsible for addressing those limitations and making that known to the public?
And how how might be the best way to do it?
Yeah, I mean, I think there are three problems that would need to be tackled. Maybe three that come to mind at least. So one is like from industry's standpoint, you're trying to sell a product, so therefore you're kind of portraying your product as like the perfect solution to any problem that some consumer might want to solve. And so the limitations aren't super obvious. And it's really up to the consumer to figure out what the limitations are or how this should be used. So I think from an industry perspective, in terms of like supplying this cloud based infrastructure for people to use, they should. I think there needs to be more careful monitoring. First of all, how they're systems being used and kind of how how could they regulate that. So like some people will always lie, right. Some people will come in and say, we're gonna use your system for this reason. And then they're gonna go use Microsoft to help build sense nets or something, whatever, like. I don't think that Microsoft necessarily knew that was happening, but that's also a little bit of an issue. So how can we solve this weird disconnect between the companies and the consumers who are using them, whether for good or not? And how can we ensure that companies do state the limitations of their products upfront? I mean, a lot of that for me comes down to like regulation and like expectations of the market. In terms of researchers who are building these things, I think it's a similar issue because we have to like portray our research as like groundbreaking. And so there's kind of this weird disconnect for me where machine learning is actually probabilistic, but the way that it's written about is in a very positive ist.
Hard science way where it's like we're proving this hypothesis and therefore our results are correct. And so that to me is a bit of. Ms. It's very misleading. So, for example, like you could have a system that works. Really well in trying to identify whether someone looks like a criminal because this you know, this paper does exist. People have tried to do this, but the moment they use like a different classification scheme, maybe it's the lighting in the picture that's wrong. Maybe it's you know, it's picking up on some some other underlying bias like race or gender. And so it's very misleading to say that like. X proves Y in this case, right? Similarly with gender classification, people will say like, well, my system is X 99 percent accurate and classifying men and women, but I could easily just go build a system that's like the exact opposite. It's like claiming that, you know, men are women and women are men. Like I can basically anyone can plug any category into a system. And so this idea that probability and like proving how probable your system is correct is factual really bothers me. And so I think there needs to be like a different method of communication in terms of like. Science communication to each other. Because right right now, it seems like it's machine learning, literature is a lot of it's less about state of the art kind of domains or a state of state of the art algorithms.
And it's more about like how much better can this one specific system perform? So I think maybe some different. Mechanisms for lake incentivizing research like this should, you know, neuron's is doing this thing now or that every paper has to have a broader impact statement. Right. Which is like a good step in a direction to say like this will impact the world in X ways and maybe this will be bad in another way. So I think trying to prove that your system is the best thing and like this is obviously the correct answer is misleading. So it makes it seem. To not only the public, but other researchers that there's like this objective truth that your system can eventually get to, which is not true. And then I also think that journalism has a responsibility for improving a bit. I think there's kind of two modes of journalism in this space, which is heavily utopian, like this is going to soar. These researchers created this awesome technology and it's going to solve all of these problems and it's super cool and nifty and they don't really talk about the researchers role. A lot of the times they actually, like anthropomorphize these systems and say, like the I learned this while actually the system was taught by people to do x ray. And then the other side is like this heavily dystopian, which makes it seem like, you know, there's like we're moving towards like this robotic overlords, singularity type future. And a lot of the time, what really bothers me and what's bothered me in talking to journalists myself is that they will write about the technology in a different way than I talk about it in my paper.
So I often refer to what I research as facial analysis technology because it's doing classification and it's analyzing people's faces. But generally, journalists report it as facial recognition, which seems like really small, but it's like a different technology, right? Like facial recognition is matching a person's identity one to one with their face. It's not necessarily analyzing gender or age or anything else. And so these little like. Tidbits of kind of misleading how technology works is not helpful. But I also think it's maybe not their fault because they're incentivized by kind of our new commercial model of journalism and getting clicks. And they also aren't necessarily, you know, journalists research a lot of things. So they aren't like super literate in what computer vision is or what, you know, speech recognition is or like all these different systems that they're writing about. And so I think. I wish there was a for that. I wish there was a better mechanism of like co-creating something with journalists. But I. I think that one's a little harder to me to tackle because, again, like I've said, I've I've tried. I feel like to to correct journalists in terms of like terms that they're using. And in the end, the article will use the simplest term that the public recognizes. And so how can we explain, like more complex ideas about technologies to the public in a in a realistic way is kind of. The path that I would see.
I I don't know.
Yeah, it's I mean, it's a the more you talk and I agree with everything you just said, the more I'm like overwhelmed almost because there's there's so much the system is so entangled in itself and there's so much of a a feeding in itself that it seems like there's a lot of folks out there who are trying to do these harm reduction strategy is right. Like like neuroses. But even that. Right. Your paper is going to get published if you're making a bigger claim about what you're proving or what you're doing.
And then you I just want to lift up, I think, an excellent point with this feminist critique actually about like, you know, the the view from nowhere, this objectivity and what what we do with that.
If objectivity is synonymous with the scientific method and if we're saying it's not, then what is science and experimentation and research become? And we could say a lot more about that. But I did have one more question for you before we closed up, which is in lieu of you mentioning the media and I think a lot of us in a certain sense fall into this trap of either utopia or dystopia.
And I'm wondering from you, can you hear a vacuum in my background? Is there a vacuum?
Will the beeping sound OK? I'll restart the question.
I did have one question for you. In lieu of you bring up the media and the utopia and dystopia framework that we sometimes fall into. I'm wondering how you would recommend listeners to separate that the hype from the reality, I guess because that's part of what we try to do in this show is really drill down to to what's real. So is there any advice that you would give to folks about how you how they would find out, you know, what's what's really happening, what's really going on?
Yeah, again, I think kind of a hard question, but I think. I would take anything with a grain of salt, I guess so, you know, if something is claiming to be perfect, it's probably not perfect because our technology is not that advanced. Believe it or not. You know, I think a lot of maybe news or also movies like Portray Technology is far more advanced than it is. But a lot of our particularly A.I. based technology is like very specific, like for very specific tasks. And I think on the dystopian side, I think it's similar where it's like, you know, the technology is not necessarily that advanced. Yes, people may be using this for bad things, but they're also probably some good things on that. It can be used for. And so I again, I I would love for people to lean into the critique of like, well, we should definitely question, for example, how police are using facial recognition. But I think they're also useful use cases of facial recognition, which I think is maybe actually rather controversial to say in like a fairness space, because they are kind of two camps. It's like we can improve this or this is terrible, we should scrap it. But I personally try to like use critique as a way to also figure out how things can be improved and constrain so that they can't be used in terrible ways. But but I'm also me and everyone else is human, so there's no way to see all of the ways something can be misused or used for good things. But I would definitely say like there, if there's a good use case, there's a bad use case. If there's a bad use case, there's a good use case. It's kind of my advice. Yes, sir.
Thank you so much for coming on this episode. Morgan, it's really been a pleasure and thank you for your really interesting research.
We're excited to see you guys.
Well, we wanted to thank Morgan so much for coming on the show today and do a little bit of a debrief. And I think the first thing that really stood out to me from this interview that I'm taking away is thinking a little bit more about the question of identity beyond just representation, but identity in all these different areas of artificial intelligence technology. So whether that's in the academy or the industry or the media. But how do we really start looking at the question of identity as not something that can necessarily be solved in a scientific sense or in a classical sense, but something that really needs to be explored to a greater degree?
So we're not taking so much for granted when we talk about, say, the gender binary. And that's something that I think we all are socialized to do to a certain degree.
And I really appreciate Morgan's invitation in his field, in his world for us to step outside of that binary and really start to critically analyze how we think about that.
Yeah, definitely. I mean, just going off of what you were just saying, I I completely agree in terms of questioning when it's really necessary to classify things. And I mean, Morgan dove into this quite a bit, so I won't wax poetic on it for too long. But I do think that it is an important takeaway to really ask, like, what are the consequences of classifying in certain cases and not even just from maybe an equality equity perspective, but also from an actual data analysis perspective. Because for me, I think about when I am creating a machine learning model or when I am trying to implement new algorithms in my own research, a lot of the outcomes of my models are really going to be dependent on what I'm on, which features I choose from my data to create the model with and the choice of a specific feature in itself. Is it classifying things? It's it's bucketing things. It's bucketing people into ideas that we have created about what is important to extract from their information. So if we are making the explicit decision to make gender binary, for example, then we are making a decision to bucket people into male and female. And that influences the models and the algorithms that are going to be making decisions about these people ultimately in society. And so I think it's important to think from all perspectives how how these decisions in what we've been taught throughout our entire lives in terms of classification, in terms of how we view identity, how they ultimately impact people throughout all the processes and all of the throughout every step of the process.
When it comes to machine learning and artificial intelligence, it really makes me reflect on what we're doing when we're building these artificial intelligence technologies and algorithms where we're really embedding our values like the human world's values into these technologies.
And so one of the first places for artificial intelligence ethics that I think Morgan is asking us to reflect on is is what are we bringing to the table? What are those biases, especially if we're never going to be, you know, bias free. All right. Hopefully, we can be a little more prejudice free. But in terms of being bias free for all bringing our biases into these technologies that we're building, then how do we do that in a way that's fair and accessible and just for people of all identities and not just the people that are, you know, in the room making these decisions. How do we make more room? Like, how do we make more space for all of this? That's something that I think I'm definitely taking away. And how important it is for us to have these interdisciplinary teams at the table who people from different perspectives who are asking very different questions. Morgan coming from a feminist and queer studies background is going to be asking different questions, really important questions that people coming from a computer science background primarily might not be asking. And although both sides are going to be asking important questions, it's going to take all of those different questions together to really create a fair and equal world.
Yeah, definitely. And I think that it even expands past this notion of interdisciplinary, but it's almost like cross domains as well, because like Morgan mentioned that he does research in academia, but he's also working with people in industry and those people in industry are impacting people who are not a part of academia or industry. And so we have to have we have to start having conversations between the people that are being impacted, the people who are creating these technologies and the. People who are researching the impacts of these technologies and figuring out what is the best way to collaborate together, to try to start to find the best solutions to some of these issues. And I think that a lot of that kind of also raises this notion that we asked Morgan, too, about who is responsible in this space, because there there is so much responsibility and risk at stake when it comes to a lot of these issues with identity. And so, I mean, we already know what Morgan's answer is now. But I'm curious, Dylan, where do you stand on this when it comes to, I guess mostly classification software, but maybe we can expand this out to the general realm of A.I. and ethics, like who do you think is is responsible in this space when it comes to making our technologies more ethical and and being building that awareness for the the layman or for the people who are most impacted by these technologies but aren't a part of the design process?
I have a very short and a cop out answer, and that is all of us. I think we are all responsible to a certain degree for the technologies that we're creating now. Does that mean that the consumer is as responsible for the racism of a machine learning algorithm and by racism?
I mean, if it's categorizing certain groups of people based on their skin color in ways that are either offensive or abusive or oppressive in some way. No, I don't think the blamers is across across the board right in that way is equal. But I do think that in so far as we all are constructing our society, you know, every day and bringing our values, the more intentionally that we can bring our values into our work, and especially for those of us with no power in industry or in the academy to create these studies, it's all about the questions that we ask and the intentions that we bring.
So we all bear some level of responsibility.
And those folks who are, you know, actively releasing algorithms out into the world. I think bear a little bit more in terms of what they can actually do to implement that. But I think it's a really live question of who bears responsibility, especially, you know, fingers crossed or maybe not cross, depending on where you fall on things. If we're moving towards a real, like, you know, theory of mind and artificial general intelligence, then the question really is, you know, who who is responsible for that? Once an artificial intelligence has a real, you know, theory of mind and is able to understand or think for itself, which is another question that Morgan raised up, like when do we use those terms of understanding or thinking to describe these technologies?
And that is that is really tough because we are painting ourselves into a corner of andthrough more fighting machines right now and that it's going to get us into some sticky territory, I think. But what about you? Just do you have a sense of responsibility? Do you agree with my answer?
I think I definitely agree with a lot of what you said for sure. I do think that based off of the research that I'm doing right now and a lot of it has to do with like algorithmic transparency as well. I think that there is definitely responsibility that should fall on the designers of algorithms to be transparent about what they're doing. From my perspective, I think that in terms of the responsibility of the algorithm being ethical itself, I think that responsibility actually lies on the consumer of the technology to understand the impact that it's going to have on them. And I think that the consumer can't be aware of these things unless there is some sort of transparency coming from the designer about how these things work. So I think it is definitely a two sided street. But, you know, everything it stems from this idea of awareness and awareness comes from transparency and explain ability and just openness, no longer hiding what these algorithms are doing, what their intentions are.
It sounds like we need to do an actual full on episode with the two of us talking about responsibility because I have a lot of thoughts about what you just said, but it is not for today.
We want to thank you all for joining us. This little teaser, a little teaser for the future.
And for more information on today's show, please visit the episode page at radical iReport.
And if you enjoyed this episode. As always, we invite you to subscribe rate and review the show on i-Tunes or your favorite pod catcher. Don't forget to join our conversation on Twitter at radical iPod.
And as always, stay radical.
Quickly and accurately automatically transcribe your audio audio files with Sonix, the best speech-to-text transcription service.
Sonix uses cutting-edge artificial intelligence to convert your mp3 files to text.
Create better transcripts with online automated transcription. Are you a podcaster looking for automated transcription? Sonix can help you better transcribe your podcast episodes. Manual audio transcription is tedious and expensive. Do you have a lot of background noise in your audio files? Here's how you can remove background audio noise for free. Sonix takes transcription to a whole new level. Automated transcription is much more accurate if you upload high quality audio. Here's how to capture high quality audio.
Sonix uses cutting-edge artificial intelligence to convert your mp3 files to text.
Sonix is the best online audio transcription software in 2020—it's fast, easy, and affordable.
If you are looking for a great way to convert your audio to text, try Sonix today.