Bonus Episode: The History that Defines our Technological Future with Archivist Eun Seo Jo
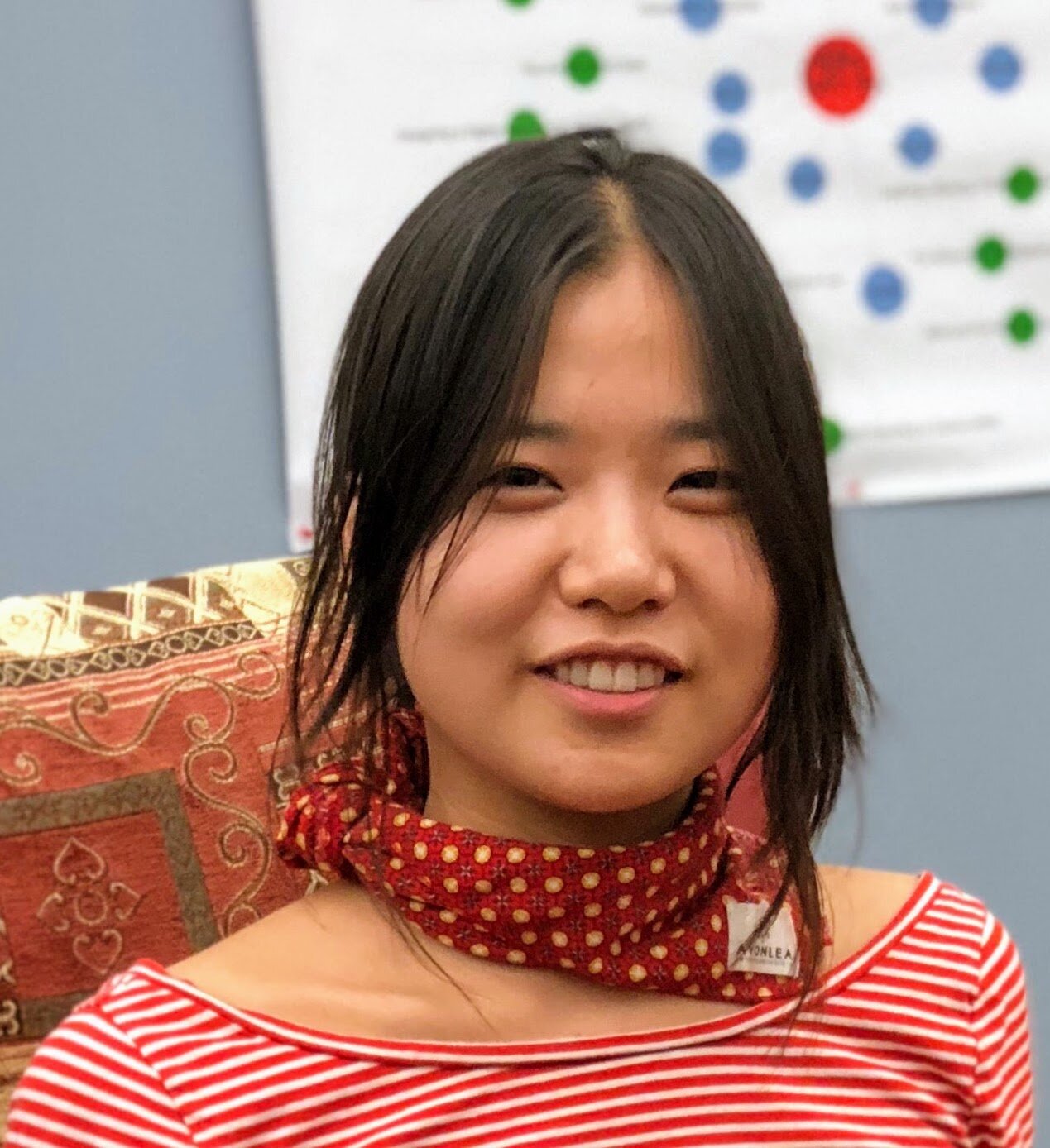
How does your data tell your story? Is historical data political? What do our archives have to do with defining the future of our technology?To answer these questions and more The Radical AI Podcast welcomes Stanford PhD. Student and Archivist Eun Seo Jo to the show. Eun Seo Jo is a PhD student in History at Stanford University. Her research broadly covers applications of machine learning on historical data and the ethical concerns of using socio-cultural data for AI research and systems.
You can follow Eun Seo Jo on Twitter @unsojo.
Relevant links from the episode:
Lessons from Archives: Strategies for Collecting Sociocultural Data in Machine Learning by Eun Seo Jo and Timnit Gebru
Disseminating Research News in HCI: Perceived Hazards, How-To's, and Opportunities for Innovation by C. Estelle Smith, Eduardo Nevarez, and Haiyi Zhu
If you enjoy this episode please make sure to subscribe, submit a rating and review, and connect with us on twitter at @radicalaipod.
Eun Seo Jo _mixdown2.mp3 transcript powered by Sonix—easily convert your audio to text with Sonix.
Eun Seo Jo _mixdown2.mp3 was automatically transcribed by Sonix with the latest audio-to-text algorithms. This transcript may contain errors. Sonix is the best audio automated transcription service in 2020. Our automated transcription algorithms works with many of the popular audio file formats.
Welcome to Radical A.I., a podcast about radical ideas, radical people and radical stories at the intersection of ethics and artificial intelligence.
We are your hosts, Dylan and Jess, just as a reminder for all of our episodes. While we do love interviewing people who fall far from the norm and interrogating radical ideas, we do not necessarily endorse the views of our guests on this show.
In this episode, we interview. Eun Seo Jo a PHD student in history at Stanford University says research broadly covers applications of machine learning on historical data and the ethical concerns of using sociocultural data for A.I. research and systems. Her paper Lessons from the Archives Strategies for Collecting Sociocultural Data in Machine Learning, discusses how the field of history and archives can positively influence the field of data science.
Welcome to our very first bonus interview episode.
That's the sound of clapping in the background.
We hope that your clapping along at home. So basically, here's here's the deal. Listeners. So before Justin, I started this podcast. I had another podcast project that was going to be in the works.
And I did a series of interviews with various folks. And again, these were solo interviews because I hadn't actually really met Jess yet. And then I met Jess and then the pandemic occurred and all these things happened out in the world. And so Jess and I are now sitting on this backlog of episodes that I recorded solo. And we wanted to do something fun with them because some of them are really cool, like this interview with. And so Joe, who as I mentioned in the interview, as we mentioned, I met at the fact conference over in in Barcelona this year, which is the same conference that I met Jess that actually originally. And so we really want to share this episode, but weren't exactly sure how. And it didn't necessarily fit our normal schedule because we want to make sure that Jess and I are both the interviewers on most of our main episodes. And so we came up with this idea for a bonus episode.
Justin not clapping.
So we're really excited to share this episode in which one so and I.
So as as just mentioned, is a Ph.D. candidate at Stanford also does some work with Dr Tim Nick Ibru, who we're gonna be releasing an episode interview with later this week and this coming Wednesday, actually.
And a lot of what we talk about in the interview is about history, about archives and about data and how we navigate ethical data. And we also talk about So's identity a little bit in her position as a woman and a Korean woman in the world of data and tech. So the way that we did this episode is a little different than our normal episodes, because I've already heard the episode, although I haven't heard it in a few months and just has never heard the episode before.
We decided to sit down and do some running commentary over the episode and we're going to see how this goes. And we would love to hear from you if you like this format or if this format annoys the heck out of you. So please use the you know, you some nice language, some constructive criticism.
But we do want to hear from you on our Twitter at Radical A I pod just started the you want to say about how this process was for you? Because we're recording this intro right after we just recorded our live commentary over the episode.
I had a lot of fun with this process. This is the first time we've tried anything like this.
And hopefully if it goes well, we can do something like this again in the future with the other backlog of episodes or maybe with other episodes that we just choose to interview people individually on once this pandemic is over and we're in person with more people, whatever it may be. I had a lot of fun and I hope that the listeners will enjoy this as well.
One thing to address going into this interview that folks should be aware of is that this was like I think like I already mentioned, one of my first interviews ever, which meant that I was still figuring out how technology worked. And this is also one of the first interviews, the first interview I'd ever done on Zune, which means that a few times over the course of this interview, you may hear a notification beep from either a text or an email that I was receiving during recording this interview because I didn't yet know how to turn them off.
So I have alleviated that. I now use, you know, nice headphones when we record because zoom. But this was part of a learning process. So, you know, if you hear a little beep. Don't worry about it. That that was. That was me. That was me, not you.
So without a learning process. Oh, we're getting proccess. But we've had we've had enough of an intro. We are so excited to. Share with you all this live commentary over this interview that I did months ago with someone who I deeply respect. So, Joe.
I'm here with you. So Joe Hune, so welcome to the podcast. Thank you. So I was wondering if you could start by just telling the listeners a little bit about yourself and where you come into this field of artificial intelligence and machine learning?
Yeah, I'm a student in history at Stanford and I became interested in AI through and through textual data analysis.
So when I first started my program, I had a lot of historical data and I was interested in using methods and compositional linguistics, mostly to analyze how the language of the State Department changed over time. And so I just I started taking a lot of classes and AI and machine learning.
And then I realized through first hand experience how machine learning practitioners were just haphazardly using datasets.
Sometimes historical data sets to train their models, which would then be used in society.
And I found that alarming and I became more interested in looking critically at the data sets that they were using.
Mm hmm. Could you say a little bit more about computational linguistics?
Yeah. So I think there's common digital linguistics. Exists as a separate field. But I think a lot of people are interested in text as as data.
So.
A lot. I think so, commendation, linguistics. As the more specific field they focus on. So all natural language processing.
They focus on specific tasks like summarization or speech recognition. Spelling, error, correction. Very, very specific tasks. But a lot of the techniques that are used for those tasks are really useful for when you're doing text textual analysis. So if you're analyzing mass sets of speeches or telegrams, anything that has.
Data in the form of text.
So that's how I became interested in an in an LP methods and apply A.P. methods on historical text data.
When you were growing up, did you. Was this the dream?
Oh, to be a historian.
I don't.
I don't know if I ever wanted to be a historian. As a child.
But I liked I liked reading and writing. But I also really enjoyed math. In fact, I think that I, I probably did better in math than in reading and writing as a child in school.
And. And so I think that I had interests in both fields.
And so this is a good compromise. What I'm doing now and it's hard it's hard to predict what jobs are will be in the future. What what what fields would be interesting or relevant.
So. Deposit it.
So what's what's interesting to me about listening to these first few questions, listening back is that I remember where I was when I began this interview. So basically, this was during finals time of the winter quarter at the University of Denver. And I was late coming out of class. And so I was late coming into this interview and I ran to the library in order to find a place to record this interview over Zoome with. And so and I went to about like probably 30 different small study rooms before I eventually found one and like the basement of the library. And then once I did not, the Internet wouldn't work. And so I ended up being like five minutes late to to this call, which I felt pretty embarrassed about. And in these first few questions, there's definitely like a level of me still trying to figure out, like recording. And then also figuring out like what questions I even want to ask because I couldn't find them at the same time. And the computational linguistics question was like a legitimate question.
I had no right to ask what it was. And I just noticed in my in my voice, like trying to come across as if like, oh, this is just for the listeners. But really, I had I had no idea what the heck we were talking about at the time. And so I really appreciated her, her conversation there. But I was also try to figure out, OK, where are we going to go with this interview? So since I still couldn't find my, like, index card of questions that I had just, you know, like what? Is there anything that stood out to you in this first? Like, few questions that I asked?
No, but that context definitely provides a little bit of clarity for me, for sure. I think that there's definitely no shame in needing to ask clarification, questions about what someone's field of research is, because I think we actually do that quite a bit in our interviews in general, even when we do a lot of research ahead of time on one end or whatever researcher is doing their research on and what field and discipline that they're a part of. I still don't really understand what it is most of the time on the Internet until I talk to them and I have them explain everything to me. So I didn't know what computational linguistics well before this. So there's there's no shame. Do you know what computational linguistics is now? Yes. I can refer you to my notes or to a nice podcast episode that details that very nicely.
Do you have a dream now in terms of like when you finish the P. D program and and all of that? Do you want to stay in academia or industry or is it just all up in the air right now?
So this is like exposing my mom.
Well, I do want to work in research. I don't know if it's in academia or in industry or in I think tank or whatever or in government. But I do want to work in research and more so and I'm less interested in in in particular tasks.
So I'm not as interested in trying to improve metrics in certain tasks, but more in.
Critical analysis of the field and policies. Something related to policy.
And the reason I ask is, is not just to put your your resumé on blast in podcast form, but is because this I mean, as we both know. Right, the field is changing so rapidly and there's so much research that's coming out right now. And I was curious if you had a sense of of where say, like your research in history and machine learning, whether that's going to create like new jobs in the future or something like that.
I think that there is there should be a new in an ideal world, and this is what my paper discusses. I think that in an ideal world, there should be an industry that is similar to being an archivist, but for data and maybe it would just be a branch of art. Art, archival studies and the archival industry or or the library industry. But I think that there need to. There needs to be.
A professional network of people who collect data and as and have that as a job because data is so important. And I think that's a type of job that I, in an ideal world, will be created for people who have language expertise for with cultural expertise, anthropologists, people with backgrounds like that. I think that they could contribute to data collection and preservation.
And that's and that's why that's why we wanted to invite you to be on the show. So I just for full disclosure, we met originally at the F eighty, now f a, c, c, t, the fact conference in Barcelona, which is about fairness, accountability and transparency in machine learning. And you were presenting a paper that you just referenced about archives and machine learning.
Could you tell us a little bit more about that paper? Yeah.
So the paper was inspired by a call within the machine learning community for some structure and guidelines for how data could be collected, because some surveys had shown that it was called, you know, it was the Wild West. There was no there was no regulation, no guidelines for how data should be collected to feed these models.
And so we brainstormed fields that we could draw from.
And one field is the archives and the libraries.
And we I think that we tend to underestimate libraries, but really they contain a lot of social socio cultural information.
They sort of they literally howse a lot of socio culture information, especially if it's language, you know, text, text data. And I think the first draft of Google was actually called the digital library or something of that sort. The Stanford Digital Library initiative. I think so. So there's a lot we can draw from a field that has been dedicated to collecting data for thousands of years. And.
We try to come up with very specific strategies and then we thought, well, all of these things vary depending on, you know, who you're addressing, the task that you're working on.
But what we can draw from are the structures and policies and the language and concepts related to data collection.
So we came up with, you know, a few strategies and we put them into categories that are relevant to concepts that machine learning, fairness. People like to bring up. So things like consent, diversity, inclusion and ethics.
So so they're they're related to policies and institutional infrastructures.
Dylan, I'm realizing on this note of not knowing things, I'm realizing that I don't know what an archivist does because I thought that an archivist.
Does work with data, but she said an archivist. But for data, what does an archive.
So it it may surprise you. Yes, but we get into that quite shortly.
I think if I remember correctly from a few months ago, I think that we answer this question exactly the movie.
Let me hit that play button then. Okay. I'm excited to find out. Can I just say that in the past section, probably one of my finest points in interviewing anyone ever is using the term.
You don't have to put your resumé on blast or not to put your resumé on blast in like a formal interview with, like a colleague. That's something that you should never do. And after I did after it left my mouth, I was like, oh, shit. Shouldn't have done that. Anyway, when my finest moments.
So specifically with working in archives, which you're, you know, as you say, has the libraries and archives have been around for a long time for a lot of human history, at least, Morgus, modern human history. What are some of those lessons or how has machine learning impacted that work in archives?
There are lessons that we can draw lessons on machine. The machine learning community can draw from archives, which is more things like how can we set guidelines for set and impose and hold people accountable for collecting data? And then and then there are also things that the library community can learn from the machine learning community about applying techniques on sorting through documents or OCR technology.
So there is a lot of benefit to go around both ways because you say more about some of those like mutual benefits between the those two fields of U.S. history and archives and computer science.
So I think also let's to start with the history side, the history and the archives side.
There's so much more information in digital format today that it's just impossible to ignore compositional methods and not just because they're available and now you can do it, but because it we're sort of at almost an epistemological change in the field for at least history and art and archives.
It's it's it's a different story. But because now you have access to all that, you know, potentially all the data on this that ever existed. And, you know, there's some bias about what exists and what survived or not. But. And now you can search through so many more documents than, you know, traditional historical methods would have allowed you one hundred years ago.
If you just walk into an archive and sort through analog, you know, hard, hard copy papers.
And so now we have to address that. Even if you're not using computational methods, you have to address the philosophical questions about what it means when you have access to all all the all of the sources related to this from that archive. So that's from his from. From the history perspective for archives.
A lot of it is related to yarding technology or techne, other technologies related to digitization and different forms of digitization.
So if it's hard copy text, then you might OCR that and then it would transform your image text into text that you can work with on your computer.
Can you. Can you define OCR for us?
Yeah. Oh, I think it's I think it stands for optical character recognition.
So it's when you have, you know, like a receipt.
So you might receive a receipt from Safeway. And then if you OCR that, then you can actually get the digits or that or the characters into computer recognized format.
So, like, if you were if you were typing it on your computer and then there's other forms like speech recognition, so maybe you have a bunch of speeches, you know, or oral histories that are archived from people who live there isn't time with interesting experiences.
And then you could. You can. You can generate scripts with that. So, like, as if you get subtitles in a movie and then you could do analysis on that.
Or even pictures, maybe their photographs, rolls of photographs from some archive.
And you can you can scan those and then they would become image data.
And even video. So there's a lot of data that could benefit from a lot of developments in the high technology.
You know, Dylan, this is really interesting to hear first, because I have no idea pretty much anything that goes on in the archivist and historian community. So just hearing what happens in the life of an archivist is interesting. But also hearing both sides of the coin is this is not something I've thought about before. I think that I always like to say that machine learning and technology should be informed by other disciplines. But I don't always have an answer as to which disciplines or in what way it can be informed. And I've never thought about how archives can inform data set curation for for machine learning. I think this is super important because a lot of people who are in like the data science community, they tend to recreate the wheel sometimes. And when we're doing stuff like trying to collect data from the world, if we recreate the wheel and we try to do that without any guidance at all from historians or from archivists, then that seems like it could be super harmful. I'm actually really surprised. I haven't heard of this before. I'm hearing about and so's work.
I think that's a great point, especially because even like for us on the show so often, we're like, yeah, we need to bring the humanities Inju technology like into these technology spaces.
I don't know if we're always as explicit as we could be about either case studies in which it's happened and it's been effective, or like specifically what humanities disciplines or least how those humanities disciplines might intersect with computer science in a positive way. And I think when Saux work and this paper that we've been referring is a great case study for a very specific way that that intersection can be very fruitful.
Yeah. And it's interesting because she talks about both sides of the coin. Like, I would have just thought that the historians should be informing data scientists and machine learning engineers. But it's interesting that she's also explaining how A.I. technology can be useful for historians as well when they're digitizing all of these really like archaic documents and big corpuses of text core by text.
I don't know if the plural of corpus got a lot of text, but when you first said corpuses, I heard a report.
This is with with AP. No, I don't think we've talked about Wales quite yet. But maybe we'll keep listening and find out. Also, we're running out of time for me as a naive interviewer to ask what an archivist is and what an archivist does, because that's the question I should have asked the very beginning of this interview.
And we're going to see if eventually I get to ask that question or not. I might have forgotten at this point. We'll find out. It's almost sounds like, you know, when you read articles about like Big Data, generally, you're talking about either like in telecommunications or image recognition or facial recognition.
But it almost sounds like we've entered this time of big data in archives as well, where because of some of this technology, we have a new way of looking at the same data. Is that fair to say? Yeah, it's I think it's fair to say and I think that there is a little bit of I think that some historians.
There were you know, I'm not I don't want to reference any one in particular, but I think there are some people who were who were sensationalizing this idea that, you know, I's taking over archives is taking over how historians are releasing information, choosing to release information and hide information, redacting documents and things like this.
But even some even the most basic things like recognizing whether this is a G or a C, that is a high technology.
And I don't know if there's any particular egregious human bias involved in that.
So so there I think that there is a lot of press surrounding problems of AI and human bias.
But some of the some of the things some of the techniques that people do work on are so fundamental and unrelated to, you know, any social biases that you can. I think that you can use technologies like OCR without particular problems.
When you already mentioned a few of the ethical considerations, at least in archives where you're even talking about, you know, what pieces of history were allowed to survive and then maybe even just naturally survive. Could you talk about more about that in particular?
Yeah. So there are a couple forms of biases that go into this. And I don't I, I think that other people probably have worked on this in in the more thorough manner than I have.
But. The most obvious are things like.
If you're taking historical data, you're taking data that represents what was the norm in the past and that Norm may not be what's acceptable today or what we want to replicate in the future.
So, for instance, the fact that we don't have we historically have never had a female president doesn't mean that that data should inform our future.
And, you know, it's it's a it's a political and philosophical debate there.
But that's an example of how certain distributions of data from the past can.
Influence how data sets make prediction or how models make predictions.
And there's there's almost a parallel there between machine learning algorithms and archives where sometimes it's described that they're both objective. Right. So, like, history is the subjective thing. And machine learning. Well, you know, the algorithm was just doing what the algorithm did, and it's just totally objective.
But it sounds like one of your critiques is that there isn't there need to be more context and that, yes, there needs to be context and there needs to be more supervision in the data that's being collected in the process of collection. Because I think that.
Most reasonable people would agree that that's on these data sets.
If they had seen the distribution of of, you know, what's what's included and what's not, what are, you know, would agree that there is something egregious problems. So I think. The problem right now is that there's not enough attention. Put into the collect the collect the data collection process, often it'll just be a grad student who scrapes a Web site.
And, you know, with as much data as they can collect as possible, that is, you know, that seems uniform on some measure.
So.
So more attention needs to be a lot of there.
So do you have any specific suggestions of how to, I guess, operationalize ethics in that?
Yeah. So we actually made a recommendation or a paper. One of them is to have a professional membership system for data collection and potentially even promote data collection as a full time career, just as archivists are.
And we're not saying archive archives are perfect. And, you know, they have problems, too. I was just, I think in The Washington Post recently about how they had altered one of the images and that had become a political issue.
But we can learn from how they have allocated resources to collecting data and then collecting information.
So something like a perfect professional membership system would force people to answer to not just their direct boss, who is often a profit seeking company, but they have their membership, their professional membership tied to an external organization that sort of oversees all of it.
So that they can't cut corners.
So a way to standardize it almost.
Yeah. So. So to have a standardized.
Standardized codes of ethics beyond the level of their direct employers.
It gets into that murky water then about, you know, regulation.
Yeah, it's always yeah. So it's not and I don't think it's perfect. I don't think it works perfectly in an archives either. And so these are just ideas and the language to talk about it, I think to to figure it out.
Yeah. Well, I know you have some experience in industry is as well, at least researching an industry. I'm wondering if these ideas, if you have gotten the chance to to test them out or to hear any feedback from folks in industry about, say, a membership system.
This one in particular is newer.
So I haven't we haven't really I think that we are working on this and we're looking forward to how this will be implemented and how the details will be hashed out.
One thing that I've heard about just in computer science and in our own articles about data and about big data is just there seems to be that that fear and that the fears are varied.
Right, from what you suggested, which is that, you know, maybe it's not even fear, but what you suggested and like the excitement of like, well, this is going to transform the entire industry of archives to the fear element of, well, it's going to take the archivist job. And I'm wondering if there are other, I guess, like myths versus realities that you deal with as an archivist and historian, working and machine learning that you could highlight for us.
Yeah, I think that there is a general fear of machine learning in general about how it'll take jobs from people.
And so I have other thoughts about it in general. But I think and specifically about archives and and history, I don't.
I think it'll be a really, really, really long time and a lot of. Dedication to the field of history in which I don't know what I don't know what would motivate that.
But for a I to take jobs from historians, because it's it's mostly a it's it's such a humanistic profession.
But I can see how archivists or librarians may have that fear of being replaced. So I think that certain tasks like checking out books or making book recommendations can be aided with a I.
But I think. I think that it would be kind of circular and self replicating. If.
I acted as the archivist because the whole point of our paper and sort of the work that we're doing in machine learning is to promote more human supervision in archival and collecting data sets for training A.I.. So.
So I hope that doesn't happen, but I can see because a lot of repetitive jobs with with seemingly repetitive tasks I think would be the first to go.
If this happens, this is such an important point. This is something that I think is so important for all data science and machine learning engineers and anyone who's involved in anything related needs to just nail down over and over and over again the fact that if we use historical data to make models, that data is never objective and it's always going to have some sort of bias, which is always going to be incorporated into the model, which will end up recreating the history in the future.
I think that is so important and something that I would like to add to this note, too, that I don't know, too, and so is planning on getting into. But when she was talking about historical data in general being a norm of the past, I think it's also important to bring up the fact that sometimes the bias of the data is the fact that there is just missing data in general. And this is something that I found to be really, really prevalent in countries that don't have good data collection practices. When I was working in South America for a little while and trying to help the government promote more open data, the fact was just that there wasn't really data out there to be open about with the community and with the public. And so we wanted to use data to help create awesome tools with machine learning and with A.I. and to help create transparency and get rid of corruption, things like that. But we couldn't because there just wasn't data there. And so that the lack of data also creates a bias in these models to the language that you use.
There was very interesting. There's not I think you said there's not good data collection and the word the word good there and putting a value on it, I think is really interesting, because even you I mean, you're coming from a very particular context or value system where you're using data for a particular means, which hopefully is like making a more equitable world for everyone, whereas like a concept like effective data collection for an authoritarian regime might look very, very different.
And which I think is part of the point that when so is getting out, which is just that data is inseparable from and especially data collection of data use are inseparable from the political and socio economic systems surrounding it. And I think it's a great point that you're making about like, well, what how do we define good data and good data collection, especially if we, you know, take for granted the old cliche that history is is written by the victors.
Right. Which is the history of, you know, colonization, all that stuff.
We're dealing with historical data. How do we separate that? Is there such thing as good data or is this just like the data that we're given? That's true.
This is making me wonder why I was never taught about good quote unquote data collection practices in my data science classes as an undergraduate student.
I think that maybe every single data science person or educator that is planning on teaching data science should probably incorporate something about archives into their into their classes.
It seems really important. I just I want to bring up what this conversation is making me think about today. So we're recording this with the days on May 29. So a few days before this episode will come out and and we're in the midst of a very interesting dialogue happening between the office of the president of the United States and Twitter and Facebook right now.
And what it means for social media, which in a certain sense is is an archive of of some of someway, some all electronic archive.
What it means for Twitter to mark the president's tweets as sensitive or as non-factual. And one of the things that so was referencing earlier was when the National Archives digitally altered a picture of the 2017 women's march, altered some of the things that were said on the science, especially that were critical of the president and some pictures of female anatomy. They altered it.
And it's interesting to think about this conversation in light of what is happening right now and in light of the changing archives of our lives. And again, this concept of truth and and post truth and who controls the truth. So even when it's like part of my, you know, more more liberal politic, it's it's interesting to think about that. Is it possible to get an archive that is the truth, as is in the same way, like an algorithm ran an algorithm? The truth as is reflecting the world as it is. And I don't I don't necessarily believe that it is. But I just really I have to say, I think it all does.
And it brings to light the fact that there is just so much power sitting with this.
So the people who collect the data hold all the power, the people who curate and. Clean the data, hold all the power. The people who use the data and feed it into the model and train the model and tune the model, they hold all the power.
And there is a lot of harm that can come from that power if it's not distributed equally. And if it's not acknowledged that, you know, it can be harmful. And I think that's just it's so relevant right now. You're you're so right.
Just did you know that one of the fastest growing industry is right now, especially for academics, is in archives and museums? And that's a really curious fact for me to think about. Like, why, why, why now? Like for a lot of our colleagues who are killing their doctor, it's a lot of them are going to end up working in museums.
And it's because there are jobs in museums to an unprecedented degree, like there are more museums opening this year than there have been in the past, like 70 years for something crazy like that.
And I wonder about that. Like, I wonder what the cultural consciousness is like. What? Why are we. Why are we doing that? Is it because of the technology that we have now? Is it because of like a new interest in preserving the past? And like, what does that mean for how the past is going to be preserved? And, like, who gets to make those decisions? I just I'm thinking a lot about that as I plan my own everyday.
Maybe there's just more data to be collected and curated and put into museums and to analyze. I don't know. This is complete speculation, but perhaps that could be one reason Per.
Interesting. Let's throw it back to.
So my sense and you're in the field, so let me know if this is wrong, is that not a lot of people know what an archivist does day to day basis. OK. So I'm just I'm wondering if. I think there's like a lot of assumptions that are made with, like what happens at a library or what happens in an archive. And is there a difference between the two? And I'm wondering if you could just break down those distinctions.
I just. I did it. I guess the whole English, that's that's twenty two and a half minutes ago with an archivist does.
I finally got there. So you will finally get your answer. What an archivist. And I will too.
Yeah. So I think there are many different types of archivists. But when we talk with the archivist we sort of mean the people who collect primary source information and that would be for us data. So there's a lot of parallels.
So if.
You're an archive. You might depending on the type of archive. But a modern academic archive, for instance, you might get collections.
So someone might have a lot of primary source documents and which in our cases is data. And they and those documents may be evaluated or praised for their value and then become part of the collection. In our case would be the dataset. And so that's what an archivist does. They they they evaluate collections. They appraise materials. They determine whether this is worth keeping in our collection. And so so that's the human element that we think is needed in in the data collection process that we can learn from.
Yeah, I mean, it seems like so if you if you break down some of the humanness in there, it would be making some sort of judgments like even if you think about appraisal, there's some sort of value system they are working on. And your sense is that that's not something that machine learning could do completely.
Oh, you mean evaluate documents, perhaps.
Yeah. Just what what is I guess the question is like, what is that human element that you're pointing to?
That would need to be done in partnership with AI as opposed to like being replaced by a.
Yeah. So I think because I is trained on on data sets, you need to have data that are that have been collected under Hice under certain supervision. Because especially if it's socio cultural data and those datasets currently are not under.
Any so often, any type of supervision, they're kind of just automatically scraped, you know, grad students that's there over the weekend and then scrapes it from the from somewhere on the Internet. And there's not even really much discussion about how or how those decisions were made when it was scraped.
What if it followed a certain format or.
Structure. So so were the bigger I guess, the the the general, the Suffield of data collection within machine learning.
We're encouraging we're pushing people to be more critical about their datasets, not just because of the ethics of Ramonet, but because it actually.
It actually helps with understanding the function and performance of A.I. models, too.
I have so many thoughts about this. First, I have to just completely agree with what Inso just said in that data sets are everything.
And if you use a broken data set, then you're going to build a broken model.
So it's in every data scientists best interests to try to have the best quote unquote. Now I'm going to catch myself using that word every time I use it. But to have the best data that you can have.
But what I was really interested in in this last part of the conversation was this idea that certain humans who are in the loop, starting with the archives, but then also with technology, are making a call, which is basically a value judgment about what data is valued in these archives and what data is worth keeping in the collection. And it's interesting viewing that from a historical perspective where I'm sure that people who have been trained for years and collaborating with big teams will make these large decisions that are, you know, checked off by many different people along the process. But when it comes to online data collection, I'm sure the process is much different and much more vague and not standardized, as you guys were talking about before. And also. There's just so much more data to be collected in so many different communities, and it's being collected by so many different organizations. There's not really any source of truth. And every company that collects its own data can just do whatever it wants. In terms of data collection, so there's just so many unknowns. There's just so much ambiguity when it comes to digital data. But there's so much more data out.
There's this it's bad in both aspects. I mean, it's good that there's more data, but it's bad that there's zero standardization.
I mean, it's it's so it's just so complex. What. What's easy what's sometimes easy as an academic is just to say, God, that that was wrong. Look what you did there, the decisions that you made about the data you collected and then used and how you used it. Those decisions were bad, decisions were wrong. And I can write like so many pages about how those things are bad. And that's important. It's important to critique.
And sometimes I think I forget that to a certain degree, decisions have to be made. Now, they don't have to be made the same way that decisions are made. And what and so is really suggesting in a very specific way, right. That we have more specific supervision of who's in the loop and when they're in the loop and the decisions being made of a human in the loop as well. It's all really important. But for these technologies to come about, sometimes you just you do have to make a decision about what data you're going to use and what data you're not going to use. You can't use all the data all of the time. And every time you make that decision, it is such a fundamentally political decision that has far reaching ramifications downstream about who it impacts, what voices are being included, et cetera. Everything that doing so saying it's just as and as you're saying, it's just it's so complex.
It's I don't know if there's a I don't know if there's a right way or a good way to do it. Maybe it's just a way to do it. That is not as bad as another way. It's like a harm reduction strategy for Dana. Definitely.
Yeah. And I mean, it's also all about that transparency, right? Whether there's a right or wrong way, it doesn't matter as long as we address the fact that whatever decision we make isn't going to be perfect and we can be transparent about it. So everybody understands that, too.
And this is why I actually think it's really important to give a shout out to to Timna to to Neugebauer, who was co-authored on this paper that we've been talking about with him. And so because part of Timbits work has been the data sheets for data sets initiative and making sure that there is greater transparency in that work, in how models work and and data being taken in.
So we'll include that link to that also in the show notes for this. But I think that the work that Timna is doing and her team is doing about data sheets for that transparency and models is really important in this conversation, too.
So. And you're also coming from a very particular socio political context as a Korean woman doing this work. I'm wondering if you would be willing to talk about how that may be shaping your research or shaping your journey in this world of machine?
Yeah, I.
I think that when I first started when I first started looking at looking at data science and competition methods for historical data, I was more interested in the techniques and the methods.
And then I just from my exposure to the culture and the people in that field, I began to see that.
Your personal also has a big impact on not just your experience, but the the science that gets created, the information that gets generated from these labs.
Know, and I think for me, my gender played a big role in seeing a different aspect of science.
And. That made me realize. And in this in this case in particular, I saw how data sets could be collected differently. But I'm sure in other aspects it's true.
I'm sure that in other aspects, people can see how gender or race can play a part in how science is shaped.
And I just saw that.
Science isn't, as you know, objective or isn't as straightforward as first principles.
And that's how I became more interested in the socio technical aspect of data science. Data science.
Mm hmm.
And part of that is probably the representation, too, of of who's at the table to make those calls is what goes into the archives and also what gets studied in machine learning.
Right.
And the agenda that people follow. So.
Yeah, it starts from the policy or it all starts from the idea and then it trickles down to the policy and then the implementation. And so I think in all of those aspects, it's.
The personal and then the social and the cultural elements play a role and technology and especially artificial intelligence, product development, say, in Silicon Valley or Boulder next to where where I am.
It has definitely gotten a rap of being a boy's club and being predominantly white as much as you're comfortable sharing.
Is that been kind of your experience? Like, have you been welcomed as a woman into that community?
Yeah, I think that the gender ratio at Stanford is a little bit better, I think. I don't know the exact numbers and, you know, I hear the numbers, but they actually honestly look different in practice anyway.
So I don't know if the numbers really matter for for for people's actual experiences, but. I would say.
Sometimes I think a lot of the biases are subconscious.
I don't think I think that most people, if they knew that this, you know, is harmful for the community or or or their students.
I don't think that they would intentionally be malicious. I think a lot of it is, you know, it's just culture or something that they're used to.
So maybe some people are just more used to seeing certain types of people in that community. And so they replicate that without thinking so.
But I think.
Part, I think the difficulty is for for women, maybe that it's harder to find.
Some engineering is difficult. And so you have a lot of it. You learn from your peers and, you know, you might go to office hours or ask your professor. But a lot of the learning comes from trying and failing with your peers. And sometimes your peers may be of a different gender. And, you know, and maybe they're less inclusive. I don't know. Not always. But and I think that's probably the biggest hurdle for women.
I mean, the question of diversity comes up all the time.
And when I talk to folks in industry, there's generally a desire to have more diversity on coding teams and research teams and things like that. And then they run into this issue where when they get that diversity, there isn't necessarily already the culture there to welcome that diversity and to maintain.
And I don't know if you have any any solutions to the history of diversity. I seem not to.
Where where? Not just gender, but any diversity hire. You know, it's kind of like a stop gap measure because it solves like a a PR crisis. Maybe it's like, oh, well, we have 20 percent women now, 30 percent women now. And and so I think that's why the numbers are really nice, because then that doesn't you know, it seems to be a really nice argument to use, but it doesn't necessarily mean the culture itself has changed.
I think that there are a lot of moves now. I think there is definitely a lot of invest or maybe not investment, but funding going into more diversity, academic intellectual diversity in computer science. And I. So I think the data science initiative is one of them. And then I think in some schools there's, you know, interdisciplinary A.I., these types of initiatives.
And I think it probably I think probably they face similar problems with hiring women to have, you know, have the gender be twenty five percent without changing the culture.
So I'm actually also part of the the Stanford Data Science Institute. And so I am one of the few humanists in the group. And I think that it will take time for people to.
Be more open to.
While one being more critical about their data sets an understanding that science is a human endeavor and that there are human biases and every step. So to understand to be open to to those criticisms.
As we move towards wrapping up, I'm wondering if you have any thoughts that you would give to students or to learners in archives or I maybe particularly women who are starting out in that field. Just general advice that you might have.
I think that my advice for women in the field in STEM would be.
To persevere and to support each other. And then for historians and other humans, I would say.
I think it's it's very important when we do research to try to find its relevance to the present world.
And.
End to end to read the news.
And, you know, because sometimes I think when I first started in my program, I was so razor focused on it, on historical questions that I wasn't paying attention to how this could be relevant to modern issues. And I think this is potentially a problem more for historians and other humanness for further human. So I think it's important to try to find how we can use our knowledge for social good.
So thank you so much for joining us today.
So just that was my interview with and so Joe and one of the first interviews that I ever did.
What did you think? Congratulations. You've grown so much since then.
Thank you. I thought I thought about, you know, 15 minutes, and I think I hit my stride. There were some good questions there. So I found myself a little bit.
No, it was great. I really enjoyed it. I think says work is really interesting to me. And I always love hearing about disciplines that can really help inform and help the field of AI and machine learning and archives. And history is one that I have honestly never really thought about. But I don't know why, because I think that it's honestly probably one of the most important fields and disciplines that could inform machine learning and data science practices. I don't know how I've not heard about this before, but I'm very glad to to now be informed about it.
Says work. Yeah, it's it's funny listening back, just like all the technical glitches and all of the like, different ways that I know I recorded it wrong and and all this stuff that that I know will probably show up also in the in the final that we release here, just how how far I've come in such a short amount of time. But also it's amazing to me like how much it betrays what I was reading at the time, which I was working up a lot on this like white accountability and tech spaces work especially around representation and especially reading the A.I. Now 2019 report, which I've we've reference before in our conversation with Sara Myers West, who's a postdoc over there.
And then also was reading a whole lot of Timit get through. And so, like, a lot about representation.
And that just came out like an accurate single question I asked. Like, every question I ask was like a leading question about representation. Like, tell me more. Mostly I want to hear what you say, but also I'm writing a paper about this right now. It's a big help. Help me orient what I'm doing in your experience.
But it's it's really it's really cool looking back on the work that I was doing. It's just it's such. It's interesting to interview people at different stages of their career and to see how they answer questions differently based on their experience and what disciplines that they straddle.
And I just think this was such a cool experience to interview someone who is still doing their doctoral work at Stanford in California at this time and place, who is straddling him being a historian and archivist.
And then this discipline of computer science is just such a particular snapshot of of this work in this world. I don't know if we always got a chance to see. So I enjoyed listening back on it, even if there are definitely some things that I would have changed.
Well, do it. It's also a snapshot of you and your work, too, right? It's a snapshot of of learning how to become a public scholar. And that's what this podcast is for both of us as well.
Well, let's hope so. OK. I like that. That is there. There's a form and function element here. We're like this is also part of an archive that we're creating.
Right. That's so meta. It's so meta. But it's it's it's right. It's like. And the choices that we're making, we're creating data based off of the questions that we ask versus the questions we don't ask. Right.
That's so true. We are data curators and our questions are political. And the way that we disseminate people's research is also political. This is actually really important. I read a paper about this earlier this week. It's in the Kaie Conference, will put it in the show notes. It's about disseminating news in or in research, in HCI news.
That's something along the lines of that for the title. I'm sorry, it just butchered the title for.
So the author is HCI Being Human Computer Interaction.
Yes. And in this paper they talk about how people who interview HCI researchers are actually at a lot of risk of spreading misinformation, because the way that you choose to interview someone can actually be political and you can ask questions that have an agenda behind them. It's exactly along the lines of this. So as an interviewer, we are archivists. We're curating and we are disseminating information to people. And we're making value judgments and choices along the lines of how we conduct our interviews and how we share this information with other people. So maybe this is a good check for us as well to to make sure that we're trying to stay as neutral as we possibly can or at least being transparent about our process.
Yeah, I mean, I really I really believe that neutrality is impossible. And I believe that every question we act, we ask is a political act. It's something that I carry around in my in my ministry to it's like every sermon I give is is political, every like form of persuasion for me, like it's a political act. And I don't mean that in terms of like we're supporting the Democratic Party or the Republican Party or whatever.
Like, it's not rooted in that necessarily.
But by political, I mean like it's rooted in power. Like, every question we're asking means we're not asking a million other questions, which means we're curating information, which means we're making a statement. So that's that's my soapbox.
The most meta conversation that we've ever had about conversations that we're having, about conversations that you've had.
Perhaps, perhaps it is. And perhaps we should save the rest of this conversation for our possible future.
Met many soon where we talk about the episode that talks about the episode, that talked about the episode of the something that was recorded. It sounds like it. But if you enjoyed this episode, we do invite you to, of course, follow along.
Make sure to follow us on Twitter at Radical A I pod and to check out the show notes for this episode, including a reference to an SOS article that we talked about a lot in this episode and other articles that we've talked about as well.
If you enjoyed this episode, we invite you to subscribe, rate and review the show on iTunes or your favorite pod catcher. Join our conversation on Twitter at radical iPod. And as always, stay.
Radical.
You can stay metter and you can be radical about your radicality right now.
It's too but it's too radical. That's the line. We crossed the line. You two met too far. I got lost.
I'm I'm in radicality inception right now.
Automatically convert your audio files to text with Sonix. Sonix is the best online, automated transcription service.
Sonix uses cutting-edge artificial intelligence to convert your mp3 files to text.
More computing power makes audio-to-text faster and more efficient. Automated transcription is much more accurate if you upload high quality audio. Here's how to capture high quality audio. Sonix takes transcription to a whole new level. Lawyers need to transcribe their interviews, phone calls, and video recordings. Most choose Sonix as their speech-to-text technology. Create and share better audio content with Sonix. Here are five reasons you should transcribe your podcast with Sonix. Get the most out of your audio content with Sonix. Are you a podcaster looking for automated transcription? Sonix can help you better transcribe your podcast episodes.
Sonix uses cutting-edge artificial intelligence to convert your mp3 files to text.
Sonix is the best online audio transcription software in 2020—it's fast, easy, and affordable.
If you are looking for a great way to convert your audio to text, try Sonix today.