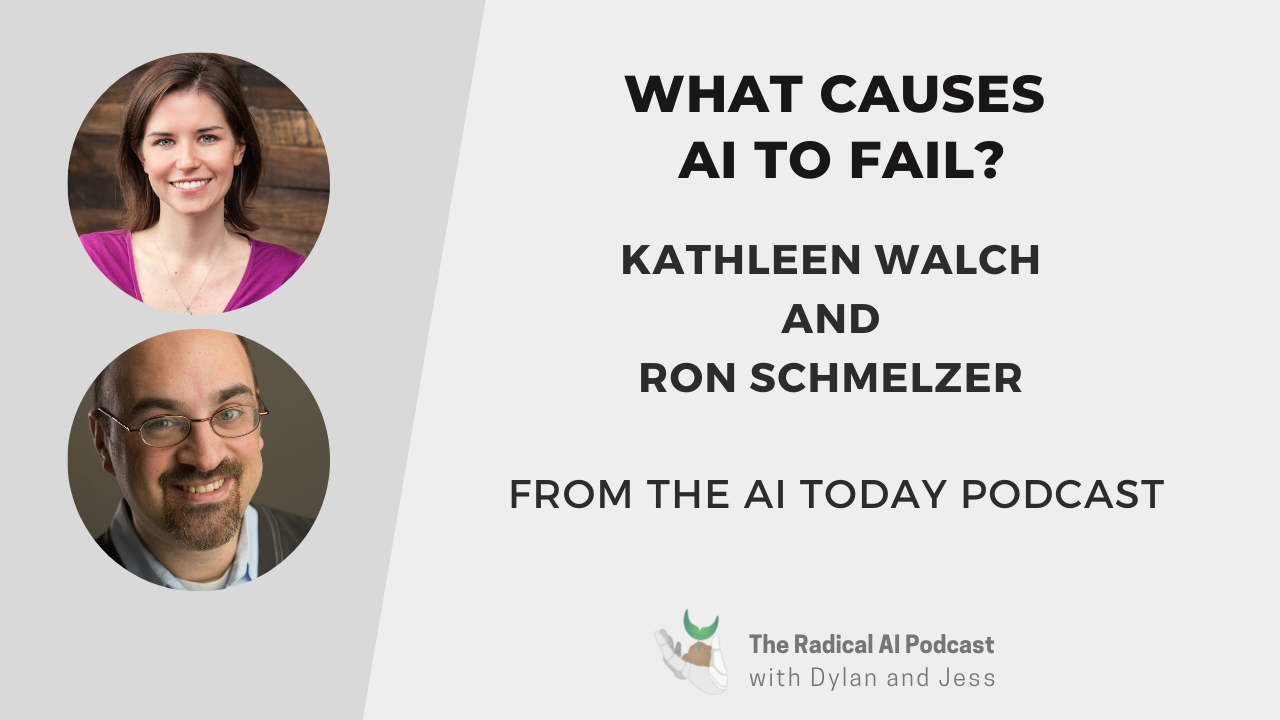
What causes AI to fail from a business/industry perspective and beyond? What metrics are used to measure and indicate failure? And how can we improve the field of AI by learning from these failures?
To answer these questions we interview Kathleen Walch and Ron Schmelzer of Cognilytica’s AI Today podcast.
Ron and Kathleen are both principal analysts, managing partners and founders of Cognilytica. Cognilytica is a research, advisory, and education firm focused on advanced big data analytics, cognitive technologies, and evolving areas of Artificial Intelligence and machine learning.
Follow Kathleen on Twitter @Kath0134
Follow Ron on Twitter @rschmelzer
If you enjoyed this episode please make sure to subscribe, submit a rating and review, and connect with us on twitter at @radicalaipod.
Relevant Resources Related to This Episode:
Transcript
AIToday_mixdown.mp3: Audio automatically transcribed by Sonix
AIToday_mixdown.mp3: this mp3 audio file was automatically transcribed by Sonix with the best speech-to-text algorithms. This transcript may contain errors.
Speaker1:
Welcome to Radical A.I., a podcast about technology, power society and what it means to be human in the age of information.
Speaker2:
We are your hosts Dylan and Jess, two PhD students with different backgrounds researching A.I. and technology ethics.
Speaker1:
In this episode, we discuss what causes AI to fail from a business and industry perspective and beyond. We ask questions like what metrics are used to measure and indicate failure, and how can we improve the field of AI by learning from these failures?
Speaker2:
In this episode, we interview Kathleen Welch and Ron Schmelzer of Code Analytica's A.I. Today podcast. Ron and Kathleen are both principal analysts, managing partners and founders of Cod Analytica. Cod Analytica is a research advisory and education firm focused on advanced big data analytics, cognitive technologies and evolving areas of artificial intelligence and machine learning.
Speaker1:
Ron and Kathleen are also both the hosts of cognitive focused podcast The AI Today podcast, which focuses on what's going on today in the world of artificial intelligence. Their episodes have easy to digest content, with experts on the subject who cut through the hype and noise to identify what's really happening with adoption and implementation of AI.
Speaker2:
And Holy Wow, just this is a special podcast swap bonus episode. For this episode, we swapped interviews with the hosts of the A.I. Today podcast, so this means that we interviewed Ron and Kathleen for this episode, and they also interviewed us on the same topic. Both of the interviews focused on the topic of failure in A.I., and if you would like to hear both of us discuss this topic in the interview that they did with us. Be sure to head over to their show and give today's episode a listen. That's right, both of the episodes are dropping in the same day, and the link for their interview of us is in the show notes. Now let's talk about failure in artificial intelligence. We're here today
Speaker3:
With Kathleen and Ron from the A.I. Today podcast, welcome you both to the show.
Speaker4:
Yeah, thanks so much for having us. We're so excited for this podcast, Bob.
Speaker5:
Thrilled to be here. I know we've interviewed you on our podcast. It was fantastic. So we're hoping to repay the favor.
Speaker3:
And for folks who are listening to this episode, the day it goes live, please also head over to A.I. today, both to show some love and also to listen to Jess. And I kind of do the reverse of this interview that we're going into right now. And so Kathleen and Ron almost to set the scene before we get into this conversation about A.I. failure, how we define it, how we see it out in the world and why it matters. Let's talk about you both and about the A.I. Today podcast. So what is this A.I. Today podcast? How did it come to be? And I guess, where is it right now?
Speaker4:
Yeah, so the I Today podcast, Ron and I started back in Twenty Seventeen, so we were over two hundred episodes right now and we really wanted to start, you know, like the name says where I is today. So we wanted to hear about use cases and how different companies and different organizations and also government agencies are actually implementing AI in the real world, what some of their challenges has been, what some of their successes have have been. And so that's what for the past four years now, going into our fifth year of the podcast, we've been focused on. So we've had tons of great interviews. We've interviewed people from across the world. So that's been that's been great. We've had guests from various government agencies, as I mentioned, international as well as us. We've had so Lord Tim Clement Jones from the UK House of Lords. We've had folks from Hungary talk about their strategy where upcoming interviewing the CTO of Scotland on their AI strategy as well. And we've also interviewed various thought leaders from Fortune 1000 companies Dun Bradstreet and Wells Fargo. You know, LexisNexis, many, many different organizations because we wanted to see how folks are actually adopting AI, what some of their challenges has been. And it's interesting, you know, there's some very broad challenges, and we'll talk a little bit later about AI failure, some of those broad, broad challenges that organizations address. But overall, I think that what we've realized is that your problems most likely are not unique just to yourself. So the more that you can talk and share and learn from others, the stronger the entire community will be.
Speaker5:
I think the other reason I'm Ron Schmelzer, also one of the co-hosts the AI Today podcast and our day job. This is actually not our day job. The thing that we spend our time doing at Analytica is we our analysts. So we spend a lot of time looking at the markets for like, you know, who are the companies building technology solutions for the space, who are the companies adopting them. And we we're realists just like you guys in your podcast. And then you mentioned that as well. Like, you know, we're optimists, we are we hope for the best in technology, but we're also realists and we know that the reality doesn't always match the hype. And that's especially the case. The thing, the thing that makes AI really so unique as a technological area, if you want to think of it that way is that there's just so much science fiction concept that the average person when they think about artificial intelligence, they have this idea in their head about autonomous systems and and smart machines and superintelligence. And they've watched too much Star Wars and Star Trek and Terminator and RoboCop and whatever it is. And they have this idea. And then but of course, the reality is that we could barely get our act together with clean data.
Speaker5:
It's like, you know, we try to make recognition systems. They have tons of problems and our conversational systems aren't so great. And that's really what we try to do in our podcast. There's lots of other great podcasts that focus on some of the research and what's happening in the future. And there are podcasts like yourselves that talk about some of the societal issues and the challenges of kind of more at this trying to put this stuff into place. And we're like, OK, all that stuff is great, and that's why we encourage people to listen to these podcasts because we don't really talk too much about them, mainly because what we're focused on is, OK, well, you know, these economists and this government agency, we're trying to do some natural language thing, and they ran into some issue where the system couldn't figure out car landed on person versus person landed on car. And those two, the difference matters quite a bit. So just a little bit of insight and background, but really thrilled. I think I think we we serve together as our podcast, podcast audiences, the broad needs for information in this space.
Speaker6:
Absolutely, and that meshing of audiences is one of the things that made us so excited to do this podcast with both of you, especially because your podcast touches on some topics that we haven't actually covered as much on our podcast. And one of those topics that we are most interested in is failure, which is, as Dylan alluded to earlier, the topic of conversation today. So before we get into the nitty gritty of eye failure, we just wanted to ask you both. I guess a primer question of what is failure in the first place? How do you both define the word failure?
Speaker5:
Yeah, that's really, really great, that's a good question. Actually, if you do a YouTube search on my name, Ron Schmelzer, you will actually find that I did a talk for Ted on failure. It's actually kind of kind of a funny talk about how we only learn from our we learn mostly from our failures and not as much from our successes because we can always pinpoint a reason or to why something failed. But we're not always sure why something succeeded. But in the context of AI and machine learning, what we have found is that organizations are trying to accomplish some goal, and they believe these organizations believe that AI machine learning technologies will help them achieve that goal could be trying to automate something and reduce their cost of their human labor costs. Or it might be trying to improve reliability. Or maybe we're trying to do something that we weren't able to do before, like take a picture of some blemish on your skin, and the system can help identify if something you should be worried about or not or like, you know, taking a picture of a plant that tells you, What is this? And these are all sort of goals.
Speaker5:
We're all trying to accomplish some goals, but usually what a lot of times what has happened is that the goals and the outcomes of what they were expecting these systems to do have not matched up with what they were hoping them to do. The end and actual outcomes have not matched their expectations. And oh, and or they've they've abandoned their projects. They start these projects and they just don't finish them because there's all and we'll talk a little about. There's actually many reasons, but it's like about 10 big reasons why. But you know, a lot of it has to do with not understanding the the challenge that is, these systems are all super dependent on data, and they can't function without good data and not realizing not getting a handle on what that what those data should be and the quality and the availability and other issues is usually what what gets people stuck for that. But I know that Kathleen has more to add on failures and failure in general.
Speaker4:
Yeah, you know, that's a great question, I think, because a lot of people don't ask that and they should be at the beginning saying, You know what? What does this look like? What does failure look like for this project? Sometimes people don't ask that question. They're not super honest with themselves, and they end up going down a path that they shouldn't, and they continue with that project for too long. You know, Ron talked about these abandoned projects depending on how much time and money and resources you've invested into that people don't always want it to fail. So they just continue to dump more time and resources and money into a failing project that doesn't always resurrect it. So you need to you need to be realistic and say, OK, if we're hitting these failure rates that we've set up, maybe we should not do this project or maybe we should start smaller, or maybe we should reevaluate what what it means and what we're trying to accomplish. So I think that organizations and anybody that's looking to implement AI really needs to to look at that and say, OK, you know, what does it look like because failure looks different to everybody?
Speaker3:
And that's something that struck me both already in this conversation and also in the conversation that we had on your show about the different stakeholders involved in those different definitions, intermingling and how difficult it is, especially for someone who's not within deep within the space to make sense of that and something that we focus on, as you mentioned, Ron, is that social element, especially like downstream social impacts of these systems. And I'm curious for you both looking at markets in general, are we seeing trends of folks paying attention to these social impacts? Or is it mostly about these projects and then the financial element and definitions of failure based off of that?
Speaker5:
Yeah, we are definitely seeing a big uptick in the all of the ethical and responsible aspects of AI. And actually, AI is kind of nice because it gets a lot of media attention. But honestly, any data driven project, even so-called traditional data project where you doing data mining or any sort of predictive analytics or algorithmic decision making where even a human might have come up with an algorithm, people are like the machine hasn't derived it on its own right. There is now a much, much greater awareness of the of of all of these issues of data and the societal need for it within a corporate environment. Certainly, there are some of these do relate to some of the business aspects of it. But I think as we were mentioning as well, there are lots of sort of non business aligned uses of AI, whether it's governmental systems trying to use AI for public health. People have become a lot more aware of of various statistics and probability, even if they don't understand it. They're like, you know, random person might be like, What's this r value? I was going to bring out that accent. Now what is what? I don't know what this means, but it's like people looking at contagion rates and they're looking at infection rates and looking at hospitalization rates, and they're recording numbers they don't quite understand, but they know how important this data is as as now. So everybody's being more aware of it. They're also a lot more in the way of privacy regulations that even if they apply overseas, it's a European privacy regulation. If you're a multinational, you're complying and you can't pick and choose. You can't be like, Well, for this population, we're not going to comply, but for this population we are, it's just too hard to do that. So they're just like, we're just going to comply because the cost of non-compliance is high. But yeah, we are seeing a lot more than Kathleen, right, when we talk a little about kind of bringing it into the process, right?
Speaker4:
Exactly. So, you know, as Ron mentioned, we've we've been doing this podcast for quite some time, but our primary focus is at Analytica, which is an AI focused research, advisory and education firm. And at Analytica, one of the reports that we published earlier this year was we we looked at ethical AI frameworks from a variety of different organizations, government agencies, collaborations as well. It was about 60 plus organizations that we looked at. And, you know, I think in general, people are starting to pay attention. Organizations are we also talk about this idea of, you know, putting ethics first, right? So you want to build these systems in a responsible way and you need to make sure that you do that from the beginning of a project. So at Cornell, we are advocates for best practices methodologies. It's really important that people do A.I. right. And so we're advocates of CPM AI methodology, which is cognitive project management for AI methodology. The first step is a business understanding step. We we get asked fairly regularly, Do you start with your data understanding or do you start with your business understanding? And we always say, well, point back to CPM AI methodology. We want to start with our business understanding, also organizational understanding. If you want to think of it that way, some people you know, government agencies, for example, may not be in business. So to say so, it's organizational understanding, and you need to make sure that you're actually solving a problem because if you're not solving a problem, don't do it. Then if you are solving a problem, that's where you need to put in your failure rate.
Speaker4:
So that kind of reps back to that first question saying, What does this project look like if we succeed? And then in the reverse, if you're not succeeding right, then we're we're obviously doing something wrong and we need to iterate on that. In addition to that, you need to look at your ethical, responsible AI frameworks, figure out how you're building this. As Ron mentioned, you can't say, OK, well, I'm going to build it one way for one one type of person, which that could be in a region or classified as a, you know, different classifications. But for other people, I'll build a difference. So we want to make sure that we're building it in an ethical and responsible way. We have seen the increase in this over the past year or two, specifically where people are really focusing on that, and we think that that's great. We want to. And you'd have these conversations because without these conversations and bringing it to the forefront, making people really aware, then it's not always that people are building things maliciously, they're just not thinking through the entire process. So that's why we say methodology, folks methodology. And you want to make sure that you are following a set of steps so that it can be repeatable so that it can be transparent so that other folks in the organization who come on board if you hire in externally or you're looking internally, understand exactly what you did and have the set of steps laid out.
Speaker6:
Absolutely, and methodology, the methodology that you just defined and the Analytica has done some great work helping put out there for the general public and for some of these engineers is a great example of doing AI right, as you were saying, and we're here today to talk about doing I wrong. And something that I'm wondering is if we're talking about failure in A.I., can we ground ourselves in some specific examples of when people have done AI quote wrong, especially possibly examples that you have both encountered on your show talking with people who have experienced failure firsthand? What are some case studies that we can ground ourselves in here?
Speaker4:
Sure. So I think it's important to also start, you know, Ron and I and the team at Analytica, we've really looked through this and said, you know, what are some of these common reasons for AI failure because you can have specific reasons for failure, but maybe that's not always the way you should look at it. It should be a little bit more broad because you can say, well, it was this one specific reason, but you know, maybe just tweak that and then it no longer fails. So we look at these general themes and say, Well, why? Why are projects failing? And general themes that we found is that AI projects are not like traditional software development projects. So when you think of them as a traditional software development project, build your team like that. You use methodologies that are not, you know, AI and data specific. Then don't be surprised if your project fails. So we have seen that as as an example, you know, and that's why we're advocates for best practices with AI methodologies specifically.
Speaker5:
Yeah. And a case in point us to bring in a specific case example, get just a little ping pong back. Here is, of course, we think about all these chat bot failures where people have approached the like, OK, I'm going to build a chat bot, right? But the functionality of a chat bot, you know, between iteration one and iteration two, it's the same. It's not like we're changing the functionality. What defines the behavior of the chat bot is, of course, the data, which is what has the chat bot been trained to do? What are its intents? We have some notable failures, the ones that have been in the press, Microsoft Tay. Of course, when don't basically don't let the internet train your chat bot, that is like, I don't even know who made that. This is not like this hasn't even even like been on Reddit. I mean, seriously, why would you let the internet train? That's just stupid, right? But you have all these smart people of Microsoft. I'm not trying to, you know, you have the smartest engineers in the room and it's like, it doesn't matter how smart you are, it's like it's a bad decision. And that all comes down to data, right? And bad training data and systems doing bad things right. But there are many other examples where Kathleen mentioned, which is the approach does a functionality thing which is like, OK, I can put this functionality in place, functionality done, check, move on. It's like, Wait a second. Don't you remember the Goldman Sachs credit card that was approving people at the same family, different spouses, different credit limits? It's like no checklist functionality not done. This is clearly data problem here. And so this is why it's like, OK, functionality, it's got to work. But what you really need to be focusing on is iterating the data because the data has the lifecycle. So that's that's number one. We got plenty of examples for you, so I'll let Kathleen keep going here.
Speaker4:
Sure. You know, and these are not in any real specific order saying that this is reason number 10 or nine or eight, why they're failing. So these are a variety of different things that we've seen. The next one is that the ROI, so your return on investment and however you measure that is not justified by the project. So at Analytica, we work with both government agencies and private sector as well. Roi can look different in both of them, and we always say, don't limit it ROI to just money, either. It can be time or resources or, you know, different, different things. So don't just pigeonhole yourself to think one thing, but it can be that sometimes hiring a person can just be faster, quicker, cheaper. If you need one hundred percent accuracy, then don't use an AI system and make sure that you're solving that business understanding. Because if you're not actually solving a real problem and it's a little toy project, then when you go to actually use this, people are not going. People are not going to adopt it because it's not actually solving anything. You also need to make sure that you have your stakeholders involved because if at the end of the day, the people that are supposed to be using this project won't, then you just build it for nothing. So make sure that you're involving them early in the process that's supposed to be in phase one because you want to make sure that they're actually going going to use it.
Speaker5:
Yes. So the usual case in point for this one where ROI mismatches expectations is usually robotics, because this is where we get into this trouble all the time. You may have seen Wal-Mart was trying to put this. Art in place for doing things like inventory, shelf scanning and a few other things, new lows as well. They have this bot that was doing this stuff and they both basically pulled them back. Wal-mart actually pulled back on its on its bot investment. Lowe's basically never really rolled it out and, you know, soft
Speaker4:
Pepper robot, too. Yeah, where you know, there's jokes in in the media now that Pepper was fired, but Pepper Robot was a robot that SoftBank Robotics had put out, and we actually had an interview on the AI Today podcast with somebody from the Smithsonian Institute in the United States. So episode number sixty seven on the A.I. Today podcast. And you know, Pepper was supposed to help attract visitors to some of these under attended galleries in the Smithsonian. Museums and pepper could help ask or answer commonly asked questions, and it would be able to be interactive. And they thought that it would bring some engagement. But what they found? You know, I don't know. They've been quiet about how successful Pepper has been that was launched a few years ago and other people have pulled out. I know that Pepper was also in
Speaker5:
A
Speaker4:
Grocery store in the UK, and actually it was a little interesting to this article that talked about it said that Pepper's experience in the real world has been checkered because actually it started to scare customers rather than engage them the way that they wanted and pepper at a lot of issues, too, because they weren't able pepper, the robot wasn't able to hear and had difficulty understanding and answering questions just because of background noise. So, you know, that's also when you build projects in isolation. Yes, obviously, you're not going to build it in like an incredibly noisy room with tons of background noise and, you know, different different situations going on. And then when so so you build it one way and when you deploy it into an actual real world situation, then you say, Oh, wait, what's going on? It doesn't understand background noise. The ceilings are about 20 feet higher than in my little test room. What's going on?
Speaker5:
Yeah, we can spend hours on this and we have like a whole lot of things, but I just want to. I want to just want to add one final point in robotics and that it's it's it's always been a checkered history with robotics. We all see those videos, Boston Dynamics, with their crazy robot running and doing parkour and flips and sort of like a little bit of the joke in the industry is like, That's great. And Boston Dynamics is awesome and making viral videos. But if they want to show their bot doing something really cool, they should show it doing something useful, like deliver pizza, you know, or, you know, mow the lawn or, I don't know, I mean, hang up, hang a picture on the wall. How about that a simple task for a human nail or put a nail and tell the robot, let's, let's see how well the bot does with that? Oh, they're never going to show that. And that's because, yeah, I mean, it's and actually, if you look at Boston Dynamics as a company, it actually itself has a bit of a checkered history. It's been sort of passed from one owner to another owner. And I think I think now it's owned by Hitachi, I think. But it was Google had it for a while or maybe Hyundai. But like, that's the thing. It just it sort of case in point to the mismatch. I mean, we can go for hours. I don't know if you want to keep going on these. There's so many, so many other reasons for for the for the failures around data quality and quantity and that sort of stuff.
Speaker3:
Yeah. And we can we can continue to to list examples. But I'm wondering if we can maybe frame these examples in the next level. So we've talked a little bit about the definition of failure or the definitions of failure, talked a little bit about those specific examples of the what. And I'm wondering if we can move into the why. So I've heard a few things that you all have said, like, there's this I for I can maybe behind that as like just this psychological excitement. Oh, this thing is new. Oh, the rest of the industry is doing it. So we need to do it just period and not necessarily think about it. I've also heard you say that like, sometimes it's not clear what the problem is, but we just kind of bring AI into it to solve for something, even when it's an undefined problem. But I'm wondering if we're seeing this right, y'all. Y'all have been doing these interviews for four years and around the time, you know, in twenty sixteen twenty seventeen there was the predictive policing and now we keep we've kept seeing these things come up over and over again. And I'm curious about why, why haven't we been able to fix this problem and it keeps coming down the pipeline or these problems, I should say.
Speaker5:
Yeah, I think there's really I think this is the unfortunate cycle with I and your listeners might might know this as well, although sometimes they don't with the whole idea of the winters, I know if that's a topic you guys have covered, but as you know, we have this sort of like inflation, this hype cycle where people get really enthusiastic, especially when we like solve some fundamental challenge in intelligence, we're like, Oh, we figured something out, we figured out deep learning or we figured out expert networks. We figured out just basic, you know, computer logic. And they're like, And then then you kind of get this like little fantasy complex, like, Oh, well, if we can do that, then we can do we can do anything. And then and then the money comes in and the government investment comes in and the researchers come in and then there's like global competition. And then we're like, Well, what we did is like, it's an onion. Intelligence is like this. This this onion. Yeah, we've unwrapped one level or a parfait. I guess if you're if you like Shrek, but it's like layers, right? And it's like we solved that one layer and we could solve all these problems, but we haven't figured out all the layers. And so we kind of hit that wall, right? And then it just so what happens is our overpromising deliver. And that's sort of like this chronic cycle with with AI. Right, right, Kathleen?
Speaker4:
Exactly. So back when we first started our podcast podcast number five, we talked about the AI winners. So if your listeners don't know about that, we encourage you to check out A.I. Today podcast number five where we talk about that and go into great detail with it. But you know, the concept of AI is not new, right? It's I mean, the term was coined in the nineteen fifties. We've gone through two winters, and so now we're back in what some people call an AI spring or an AI summer. We've had this resurgence and we can say, Well, why right? Why back in the nineteen fifties did we start doing all this great stuff took us to about the nineteen seventies, and then we had our first winter, came back, had our second season with A.I. and expert systems back in the eighties and the early nineties. Then we went into another decline and another winter, and now we're back. And why? Why even go through the declines and why have you know, why have we not maybe advanced as far as we think that we should have if it's been around since the nineteen fifties? And I think the overarching problem and a big reason for failure is that we overpromise and under-deliver on what we can do. So back in the nineteen fifties. Think about how much data we had, not merely what we have now, right? Think about the compute power, not nearly what we have now. Well, if we want these systems to do all these great things and we didn't have all of that that was needed to to make things move forward, but we have the ideas. Well, then you can see how we're overpromising on what we can actually do and then under-delivering on it.
Speaker4:
Same thing happened in the second wave of AI. Expert systems were really brittle and people said, You know what? We're investing all of this money, time, resources, and at the end of the day, it's not really giving us the advantage that we need. It's not really moving us forward past what humans can do themselves. And so we had a pullback in investment and had another winter. And now we're in our third wave and we've been able to accomplish some really incredible things. But you you need to make sure that you manage expectations and really understand problems. I can solve problems I cannot solve and then set your expectations and make it realistic. And I think that we continue, you know, Ron mentioned at the beginning of this podcast where we have the science fiction idea of AI in the science fiction idea of robots and what we think intelligent machines can do. And that is not always where we are. In fact, it's not where we are, right? I mean, like we do not have super intelligent machines. Think about Westworld. We do not have robots that look just like humans that we can shoot and kill and then come back the next day. Like in Westworld, we do not have that. So if if that's what science fiction is telling us, and then we go, but we don't have that what we're overpromising and then obviously not being able to deliver on that. And I think that people can quickly get underwhelmed with what we can do rather than saying this is what we can do. Let's expand on that instead of reaching high, high to the sky and then not being able to meet those expectations.
Speaker6:
Let's follow the thread of thought, actually, because Kathleen, when you were talking about us being in an eye spring or summer right now, I was thinking in my head, Oh no, winter is coming and I was trying to think through, you know, what could cause that winter to come? And so I'm wondering if through these interviews that you've all conducted over the last few years, do you have a sense of in what ways right now as a discipline, I is under-delivering or overpromising?
Speaker5:
Yeah. And you know, actually, it's actually it's a good question as to what season we actually are in. I mean, some could say that we actually are starting to see signs of entering a fall. It's possible we are not in a winter. Obviously, the winter would be no more investment and no more interest and poor researchers going finding other things to research that that's what that's, you know, we're in the winter and that's happening. And you say you bring up neural networks and they'd be like, don't don't mention neural net. That's when you know, when you're in winter. That's what happened. By the way, in late nineteen nineties, if you brought up neural networks, you'll be like, Don't talk to you about neural nets. So but there is a lot of indication that we are in a bit. We may have we might have actually plateaued in the cycle, mainly because we're now starting to see more acquisition activity, more consolidation activity in the vendors. We're starting to see some pushback on on the promises of AI. We know that there's a number of actually articles that have been on the press and actually articles that will probably be coming out in the press talking about how, like, for example, there was article about how IBM Watson totally missed the mark. All their promises about Watson Health did not really materialize. The challenges of actually making a work in the public health sector is actually very hard. And the interesting thing is is like in our interviews that we have in a number of podcasts and maybe by the episode numbers.
Speaker5:
But we've talked to people in the health care industry and they say, Well, we're actually some of the most conservative adopters of technology. I don't know why people thought we would have been the first to adopt. We're usually one of the slowest, you know, to adopt. You know, we have a lot of regulation that I have to go to clinical trials. It's a very well proven path of trying to introduce new technology, especially when you're trying to do diagnostics or therapeutics or anything like that, right? But IBM charged headfirst into that ran into all these problems as they as was expected and then pulled back. And then I think I think we're starting to see I don't know if you guys may see it, but we are starting to see some sort of like sort of the Silicon Valley model, if you will, of building tech companies is starting to get some pushback because there's a lot of this fail fast and break things and don't really care about the users. And you know, if you're not the customer, you're the product. It's becoming a lot more cynicism about that and people not trusting Facebook, which probably is a good idea not to trust companies like Facebook. But then if you look at Google, I mean, it's not that I have anything in particular about Google, Facebook, Microsoft, any of the FAANG, right? But look at the AI researchers who are the top AI researchers.
Speaker5:
Yann LeCun, he is at Facebook, right? You know, you have the DeepMind folks, part of Google. You have other folks, part of Amazon, part of IBM, and then you're like, OK, they're trying to solve these AI problems. But within the context of a company, if in the case of Facebook, whose primary job is to monetize your data, right, monetize your privacy. And it's like, should I trust them? The answer is no. Of course you shouldn't trust them, right? And and so and so that's actually starting to have some impacts on on AI adoption. People like I don't know if I, you know, don't trust the algorithm on this sort of stuff, which is which is I think I think it's fair. I mean, we are we are just like, you guys are very pragmatists here. It's like, no, there's no reason to generically trust an algorithm. But are we going to start seeing abilities to consent? Are we going to start to see ability disclosure even? Can you even opt out? And I think that's kind of where where there's a lot of, I guess, agitate or not. The right word is, but there's a lot of sort of concern that it may or may not be possible to to truly opt out because opting out might mean cutting off something that's incredibly important in your life, whether it's a payment system or a transportation system or a health system or a finance system or an education system. These aren't things you can really opt out of.
Speaker4:
No, you know, at Analytica, too, we always say, start small, think big and iterate often. And what we've seen is that some companies. Think big and start big and then wonder why it fails, so, you know, we always say, you know, as Ron mentioned with IBM Watson Health, I mean, that's an incredibly difficult problem to solve. And also, there are certain industries that just are more risk averse than others. We've gone to some, we've spoken at construction conferences and they are a pretty, you know, technologically risky, risk averse industry. And I is not they are not a quick adopter of AI. They're a laggard in that space. So don't go in and then think that you are going to be so disruptive and totally revolutionize the industry when people are are resistant to that. So if you think big but you start small and you iterate often, then you can start to see more successes as well. And talking about health care in particular, you know, we've had a few interviews with some great people. Podcast number one seventy four was about more AI in pharma. We had Subrata Mukherjee, who's the head of innovation and emerging tech at GlaxoSmithKline. We've also podcast one sixty seven was our interview with CVS Health and then podcast One Ninety One. We interviewed Ellen Kazi, who's the who's with United Health Care Group and all of those, you know, people from many different companies within that very large, you know, health care pharmaceutical industry they are risk averse to because there's a lot of regulations in place and laws and regulations that you need to make sure that you're following and you don't want to just jump into innovative technology when you haven't fully thought it through.
Speaker4:
So, you know, I respect that. I think that that that's great. You know, don't don't just jump into something and not fully think it through. We've seen the implications of that. We talked about, you know, chat bots, things like that where you can go rogue and and you don't always get the outcomes that you want. So I think you need to understand that to, you know, understand what industries really do push push the needle forward, which ones don't and use that as well. Otherwise you're you're going to start seeing that. We're just overpromising on what we can do and then under-delivering on it. And I think that we saw that with we we always point to IBM Watson, their health. But I think that because that's something that was very easily seen. Also, the media likes to point out all of the failures, and they don't point out the successes or the boring, good, mundane use cases that that really are helping to move things forward because that just doesn't make a great news article.
Speaker5:
Do add one small thing on this over promise and deliver a good sort of case in point of this is sort of Tesla's full self-driving right, which is that, no, we haven't even achieved autonomous vehicle driving, especially a very hard problem. And if you talk to researchers like Rodney Brooks, they'd be like, This is like one of the hardest problems you're dealing with literal randomness that can happen. You know, dark conditions like conditions, rain, snow people, random objects. It's like, this is like one of the hardest problems. But you have people like Elon Musk going, We're going to be full self-driving within blah blah blah. I'm out of time. Give us your $10000. We'll upgrade your your Tesla vehicle. And the whole time, I think to myself is like, why don't you think big and do what we said? Think big start, small iterate. Often you don't have to promise full self-driving. Give me like we will keep you in your lane. We'll do autonomous lane, automatic lane keeping or we'll do what's called adaptive cruise control. So if you set the cruise control but something's coming, these things are doable, right? Or it could be things like advanced warnings and this and that. Or, you know, maybe maybe routing and facilitate your I've seen things sort of like help you parallel parking. There's a lot of people who can parallel park. Nothing wrong with that.
Speaker5:
That seems to do OK because it's a very controlled environment. But no, we have these like crazy crazy claims. It's like, what do you expect? It's like people are going to hop into the backseat, push the full self-driving mode and they're going to run into a tractor trailer. And it's like not much of a surprise. And so we're like, I don't really understand, I think, why there's such a necessity to have to jump to to the to the far, far acclaim. And of course, you could say, yep, could be a profit motive, could be trying to sell more vehicles, it could be trying to sell the upgrades. But I'm like, Dude, you'd sell just as much. People are not driving Teslas because of the full self-driving. They're driving because it's an electric car. And this is not those things like, I wasn't going to do it, but full self-driving I'm in. So, yeah, it's a lot of weird misalignments. I think on this, a lot of other things. We don't understand what the motivation is for neural link, the whole brain human. I understand if you were like handicapped, paralyzed, using that to facilitate that would be fantastic, but that's not what they're doing. So I don't know. Alan, call us. We'll talk to you on our podcast. We'll talk to you here. We'll do a joint podcast with radical AI. How about that?
Speaker3:
So let's let's. As we move towards closing, I'm just going to ask a personal, blatantly unfair question, which is moving into that success space. I'm curious for you both individually, so not necessarily in terms of the market definitions, but what does success look like if there is like a successful ideal world of artificial intelligence? Is it that like 1950s vision? Is it the world in which we can promise big things and then create big things through this? What does that look like? And if you'd be willing to say, like, how would you measure that?
Speaker4:
Yeah, that's a great question. And for me, what I think would be successful, I application and adoption is this idea of augmented intelligence where we're not replacing the human, but we are helping the human be better at a certain task or role. So I look at this as I do not speak multiple languages, but I like to travel when we can. So wouldn't it be great if I could go to France or Indonesia or Japan anywhere in the world that does not speak English and be able to just freely converse with them? And they would understand me and I would understand them thanks to the power of AI. So you're not replacing the human, but you're just helping me be a better human also with, you know, doing certain tasks better. Wouldn't it be great if you had that augmented intelligence next to you to say, Hey, you know, I'm an accountant? Tax law just changed. Hey, do you remember this? Oh, maybe you should look at this. Thank you. Now you help me find my taxes a little bit better. So, so things like that where you're able to just, you know, be a better human, I guess, because of AI. That would be something that I would really I would consider success.
Speaker5:
Yeah, I think that's a great place to start. I mean, I mean, basically, as I say, great technologies are almost invisible. It's like, we don't it's not this jarring thing which is sort of imposed upon us. It's useful because it helps us in our life. And that's really what most technology has been throughout the whole human evolution. We've invented different things from vehicles to, you know, machinery and equipment, and each time we've done that, it's actually helped make our lives better. It's it's expanded our quality of life. It's allowed us to live longer, healthier lives, be more prosperous, you know, and that's really well, that's what makes the technology successful. Technology is not successful when when it when it does the reverse, you know, when it makes her quality of life worse or if it makes something we've done harder. You know, that's not what we want, you know, from from any sort of successful technology and kind of where we are right now to sort of set those expectations were we actually are with artificial intelligence. A lot of this is not as much an artificial intelligence as much as it is.
Speaker5:
We are finding better ways to derive more insights from data and we have machine learning that helps us derive those insights so that someone doesn't need to program it and code these hard code rules. We can do some discovery and what are the situations in which that is really very helpful. So, you know, I am just like Kathleen. I'm very hopeful that sort of in this evolution, this wave of a of AI that we've had, we've we've been able to to be much better about that, be more thoughtful and AI. But I do think that the world is becoming more aware of sort of their data footprint of of sort of the responsibility that organizations need to have about data treating data like like it's it's a really important asset in treating our what's called agency as humans, you know, to be able to to have freedom of choice and to be able to sort of live without impedance, I think that's becoming more and more important. I hope it's going to become increasingly easier over time. But you know, we'll have to see how the how the world evolves.
Speaker6:
I'm impressed that we were able to take a conversation that was centered around failure and end with hope, that's something we love to do. All the Radically podcast is end with an optimistic lens on the topics we're talking about, and unfortunately, we are out of time for today's conversation. But if you would like us to hear us talk about these topics extensively for another hour, be sure to check out. As Dylan was saying before our podcast swap on the I Today podcast, where we get into some of the more ethical issues and nuances of this problem that we're discussing. And for now, Ron and Kathleen, we will be sure to include many links to your show and the various episodes that you mentioned in our show notes, but for this conversation. Thank you so much for coming on our show and doing this podcast. Walk with us and for being here today.
Speaker5:
Thank you so much for having us.
Speaker4:
Yeah, thanks so much. We had so much fun.
Speaker2:
We want to thank Ron and Kathleen again for a wonderful conversation about failure in artificial intelligence, and this was fun for us to do because so many of our conversations on this show are rooted in how we define or measure or critique or explore success in artificial intelligence. And so this was a slightly new framing for the show. And just what do you think? What was your primary takeaway from this conversation with Ron and Kathleen?
Speaker1:
Oh yeah, we love talking about failure. Talking about failure is great. As academics, I think we love talking about critique. We love talking about things that are wrong. We love picking things apart, and failure definitely falls under that umbrella. No. But actually, this conversation, I think one of the things that stood out to me the most was actually when Ron brought up the I winters, I realized that I. I've heard that expression many times, but I actually never knew what an AI winter was. And so he was like, Yeah, you guys talk about this on your show, right? And I was just kind of it was like one of those like fake nods where you're like, Totally, Oh, huh. We've definitely heard of this before. And in my head, I was like, Wow, I actually am glad that you're explaining this because I did not know what this was. And I thought it was one of the reasons it stood out to me was because Ron mentioned that in an AI winter, there is no more funding for AI because the hype is gone. And so nobody really wants to be responsible for funding something that nobody's really excited about. Or maybe that doesn't have any promise anymore. And I just pictured NSSF having no more grants available for people researching AI. And then I pictured my potential for getting money, for researching AI and being able to be paid for my research. And that was a scary thought. But hopefully, hopefully, even if we are entering an AI fall or whatever phase, whatever season AI is currently in right now, hopefully that doesn't impact our potential to do research on this kind of stuff in the future. That was just, I mean, kind of unrelated to the conversation. Totally, but that actually was my immediate takeaway. What about you, Dylan? Yeah, no.
Speaker2:
I like I like the conversation about winters. I think sometimes in our show, partially because there's a lot of great podcasts out there that are focusing in more on the industry side, whereas we you include the industry side. But to talk more about social phenomenon, I feel that it was interesting taking the kind of industry first perspective here, which includes this history of AI winter, especially thinking about investment and like where the money is going. And it made me think about the cyclical nature of technology development, especially as AI has been concerned, because it's always been this dance of, OK, well, we have this dream of what AI is, and this is going back into what, like the fifties sixties of like we have this dream of what this is now. Do we have the technological capacity? And I think sometimes we tell the story now that like, Oh no, we actually have the processing power to do the things that we've always dreamed of doing. And to some degree, we do, at least to a greater extent than we have before. But in a lot of ways, there's still a lot more that can happen in the future. And so maybe this is the next like false summit where we think that we're living in the now where, hey, I can solve everything or whatever. But but at the same time, right, I'm not trying to say that we should stay in that narrative because that's what it is for me is like, what is the narrative we're trying to tell here? And then I think that drives some of the where the finances and resources are and hype as well.
Speaker2:
I think the hype is key here, actually, and the narrative and the narrative around that hype, because to some degree, and this includes business, you know, it's about storytelling, it's about what are the stories we're telling and then how are those resources allocated based on the stories that we're telling and the systems that we have in place? And so I think this is important conversation again, even in that reframing of going from thinking about, OK, well, what does success mean? What is this like overpromising? We're going to solve all the world's issues with AI, like maybe even that framing is doing us a disservice or potentially causing harm. Like what if we start from maybe not like using the term of failure or the narrative of failure? But what if we start from a place of humility, a place of, well, we don't know everything, a place of, well, maybe let's just not promise anything, but just like kind of see what happens? And, you know, maybe that's not great for the bottom line or or a certain companies because there are these capitalist system that we're in, right? But I think that reframing could be very helpful for a lot of us trying to figure out how to do artificial intelligence and by do I mean design and implement artificial intelligence and ethical solutions, you know, how do we reimagine this? And hopefully that's one of the hopes that I got from this conversation is an invitation for that.
Speaker1:
Yes, I love the language of reimagining, and it brings me to redefining which actually, it makes me think of the first few episodes, honestly, probably the first year of episode. Loads of this podcast, I think every single episode that we did, at least one of us mentioned something about how language is power. We were really hyper focused on that theme. That language is power. And this is making me actually think back to that, that concept a little bit because I think you're totally right. The way the language that we use around this is actually really important in the way that we frame not just the failure of AI, but the way that we frame the success of AI in the way that we even frame the goals of AI. And the motivation behind why we're building an AI system is really important for how it can be successful or how it can fail. And so for me, I'm thinking of how in businesses and industry settings, usually the way that the goal of AI is framed is for it to solve a problem. And so we go in with that mentality that the AI, if it's successful, will solve that problem. And if it fails, it will not solve that problem.
Speaker1:
But maybe that language is actually limiting and is the cause of failure in itself because a lot of problems can't be solved. And how do you even know when something's been solved versus not? I mean, as humans, I feel like we struggle with that regardless of if technology is involved. So I really liked Kathleen's framing, or at least the word the language that she used, which was augment. And actually, I think that the way that she defined successful A.I. is like almost spot on with the way that I define it as we've been doing this measure mentality series and talking about success in AI so much. And I do think that if we were to frame AI, the goals behind AI differently and instead of saying the AI is here to solve a problem for us saying that it's here to help humans solve problems or it's here to augment human decision making or something along those lines, I actually think that it would help us be more successful with our AI more generally. And maybe this is all just semantics. Maybe nothing would change except the way that we talk about this stuff, but it's still intrigues me.
Speaker2:
Yeah, I just I completely agree. And if you all would like to hear more of our thoughts on the topic of failure and artificial intelligence, then please check out the podcast swap that we did with AI today, where they interviewed us around very similar topics and very similar questions as what we covered with them in this interview. Again, that link to their episode with us is in our show notes, and that's all the time we have for today. So for more information on today's show, please visit the episode page at radical A.I. dot org.
Speaker1:
Also, just a side note that if you're looking for the show notes on our website and you can't find them whenever we interview more than one person at a time that is actually in our Community Panels section of the show notes. So if you scroll all the way down on our home page of radical A.I. to org, there you will find all of our panel discussions. If you were ever wondering and confused and if you enjoyed this episode, we invite you to subscribe, rate and review the show on iTunes or your favorite pod catcher. Catch our regularly scheduled episodes the first Wednesday of every month with some bonus episodes like this one in between. Join our conversation on Twitter at Radical i-Pod. And as always, stay radical.
Sonix is the world’s most advanced automated transcription, translation, and subtitling platform. Fast, accurate, and affordable.
Automatically convert your mp3 files to text (txt file), Microsoft Word (docx file), and SubRip Subtitle (srt file) in minutes.
Sonix has many features that you'd love including enterprise-grade admin tools, world-class support, collaboration tools, share transcripts, and easily transcribe your Zoom meetings. Try Sonix for free today.